0001471781
false
0001471781
2023-08-28
2023-08-28
iso4217:USD
xbrli:shares
iso4217:USD
xbrli:shares
UNITED
STATES
SECURITIES
AND EXCHANGE COMMISSION
Washington,
DC 20549
FORM
8-K
CURRENT
REPORT
Pursuant
to Section 13 OR 15(d) of the Securities and Exchange Act of 1934
Date
of Report (Date of earliest event reported): August 28, 2023
GBT
TECHNOLOGIES INC.
(Exact
name of small business issuer as specified in its charter)
Nevada |
000-54530 |
27-0603137 |
(State
or other jurisdiction of incorporation or organization) |
Commission
File Number |
(I.R.S.
Employer Identification No.) |
2450
Colorado Ave., Suite 100E, Santa Monica, CA 90404
(Address
of principal executive offices) (Zip code)
Registrant’s
telephone number including area code: 888-685-7336
Check
the appropriate box below if the Form 8-K filing is intended to simultaneously satisfy the filing obligation of the registrant under
any of the following provisions (see General Instructions A.2. below):
☐
Written communications pursuant to Rule 425 under the Securities Act (17 CFR 230.425)
☐
Soliciting material pursuant to Rule 14a-12 under the Exchange Act (17 CFR 240.14a-12)
☐
Pre-commencement communications pursuant to Rule 14d-2(b) under the Exchange Act (17 CFR 240.14d-2(b))
☐
Pre-commencement communications pursuant to Rule 13e-4(c) under the Exchange Act (17 CFR 240.13e-4(c))
Indicate
by check mark whether the registrant is an emerging growth company as defined in as defined in Rule 405 of the Securities Act of 1933
(§230.405 of this chapter) or Rule 12b-2 of the Securities Exchange Act of 1934 (§240.12b-2 of this chapter).
Emerging
growth company ☐
If
an emerging growth company, indicate by check mark if the registrant has elected not to use the extended transition period for complying
with any new or revised financial accounting standards provided pursuant to Section 13(a) of the Exchange Act. ☐
Securities
registered pursuant to Section 12(b) of the Act: Not applicable.
Title
of each class |
Trading
Symbol |
Name
of each exchange on which registered |
Not
applicable. |
|
|
Item
7.01 Regulation FD Disclosure.
GBT
Technologies Inc. (the “Company”) from time to time presents and/or distributes to the investment community at various industry
and other conferences as well as through press releases, slide presentations to provide updates and summaries of its business. A copy
of its abstract describing A Qualitative Study That Explores the Implementation of Artificial Intelligence in Integrated Circuit Design,
as submitted by Danny Rittman, the Company’s CTO as a dissertation presented in partial fulfilment of the requirement for the degree
of doctor of computer science, is attached to this Current Report on Form 8-K as Exhibit 99.1.
The Company undertakes no obligation to update, supplement or amend the materials attached hereto as Exhibit 99.1.
The
dissertation will be published in the next upcoming weeks on ProQuest – an Ann Arbor, Michigan-based global information content
company that provides applications and information services for libraries, providing access to dissertations, theses, e-books, newspapers,
periodicals, historical collections, governmental archives, cultural archives, and other aggregated databases.
The
information in Item 7.01 of this Form 8-K, including Exhibit 99.1 attached hereto, shall not be deemed “filed” for purposes
of Section 18 of the Securities Exchange Act of 1934, as amended (the “Exchange Act”) or otherwise subject to the liabilities
of that section, nor shall it be deemed incorporated by reference in any filing under the Securities Act of 1933, as amended, or the
Exchange Act, except as expressly set forth by specific reference in such a filing.
Item
9.01 Financial Statements and Exhibits.
(d)
Exhibits. The following exhibits are filed with this Form 8-K:
SIGNATURES
Pursuant
to the requirements of the Securities Exchange Act 1934, the registrant has duly caused this report to be signed on its behalf by the
undersigned, thereunto duly authorized.
|
GBT
TECHNOLOGIES INC. |
|
|
|
By: |
/s/
Mansour Khatib |
|
Name: |
Mansour
Khatib |
|
Title: |
Chief
Executive Officer |
Date:
August 28, 2023
EXHIBIT 99.1
A qualitative study
that explores the implementation of artificial intelligence in integrated circuit design.
A Dissertation Presented
in Partial Fulfillment of the
Requirements for the
Degree of
Doctor of Computer
Science
By
Danny Rittman
Abstract
The development of data processing technology
relies heavily on integrated circuits (ICs), which are electronic components that perform various tasks, including complex computations.
Designing and manufacturing these ICs is a challenging and expensive process, as the pursuit of higher performance, miniaturization,
and energy efficiency drives the industry forward. While artificial intelligence (AI) is expected to handle the task, technological
limitations persist. This qualitative research purpose is to investigate the gap between AI applications and IC design by exploring
available options. The study adopts a qualitative approach based on the interpretivism paradigm and involves interviews with eight
industry professionals with extensive expertise and experience in AI and integrated circuits. Journal articles on AI and IC design
were also consulted to supplement the participants’ insights. Evidence suggests that AI is revolutionizing the computing
industry through the use of advanced ICs. Future microchip development will continue to incorporate more AI systems and methods
as a core part of design automation. The integration of AI is expected to bring various benefits, such as the efficient automation
of repetitive tasks, handling large IC design and verification processes, addressing complex computational tasks, and exploring
new possibilities. The study’s findings emphasize the immense potential of AI technology to propel the IC design industry
forward, allowing for advanced microchip design and unlocking numerous possibilities for future technological advancements.
Keywords: Artificial intelligence,
integrated circuits design, IC verification, Computing power.
Table of Contents
Chapter 1: Introduction |
1 |
|
|
|
|
Study Problem |
3 |
|
|
|
|
Study Purpose |
4 |
|
|
|
|
Research Question |
5 |
|
|
|
|
Conceptual Framework |
5 |
|
|
|
|
Significance of the Study |
7 |
|
|
|
|
Researcher Positionality and Reflexivity |
7 |
|
|
|
|
Delimitations and Limitations |
8 |
|
|
|
|
Definition of Terms |
8 |
|
|
|
|
Chapter Summary |
9 |
|
|
|
Chapter 2: Review of the Literature |
10 |
|
|
|
|
Literature Search Strategies |
10 |
|
|
|
|
Artificial Intelligence |
11 |
|
|
|
|
Integrated Circuits |
13 |
|
|
|
|
Artificial Intelligence and Integrated Circuits
Design |
14 |
|
|
|
|
Circuit Verification |
18 |
|
|
|
|
Gaps in the Literature |
20 |
|
|
|
|
Conclusions |
22 |
|
|
|
|
Chapter Summary |
23 |
|
|
|
Chapter 3: Methodology, Design and Methods |
24 |
|
Research Methodology and Design |
24 |
|
|
|
|
Population, Sample, and Participant Recruitment |
25 |
|
|
|
|
Data Collection Instrumentation and Procedures |
26 |
|
|
|
|
Data Analysis Procedures |
27 |
|
|
|
|
Trustworthiness |
27 |
|
|
|
|
Ethical Assurances |
28 |
|
|
|
|
Chapter Summary |
29 |
|
|
|
Chapter 4: Findings |
30 |
|
|
|
|
Description of the Study Sample |
30 |
|
|
|
|
Results |
31 |
|
|
|
|
Discussion of Study Findings |
37 |
|
|
|
|
Chapter Summary |
37 |
|
|
|
Chapter 5: Discussion and Conclusions |
38 |
|
|
|
|
Limitations of Study Findings |
38 |
|
|
|
|
Interpretation of Study Findings |
39 |
|
|
|
|
Practice Implications of Study Findings |
40 |
|
|
|
|
Researcher Reflections |
40 |
|
|
|
|
Recommendations for Further Research |
41 |
|
|
|
|
Conclusion |
41 |
|
|
|
References |
43 |
|
|
|
Appendix A: Questionnaire |
56 |
List
of Figures
Figure 1: Conceptual Framework |
6 |
|
|
Figure 2: Question 11 results analysis |
34 |
|
|
Figure 3: Question 12, 14, 15 results analysis |
35 |
Chapter 1: Introduction
Technology is an ever-changing field that
seeks to solve complex problems with machines and computers (Liu et al., 2016). While the invention of computers kick-started the
era of the modern technological world, there are still areas that are moving much slower. Advances such as artificial intelligence
(AI), machine learning (ML), and powerful computing devices, have altered the handling of different activities. Technological solutions
currently cover almost all areas, with a unique adaptation of computational solutions in each field. The expectation is that technology
will keep on improving problem-solving, with some ventures seeking more efficient machine coordination in the solutions. The mentioned
advances in technology all rely on core components called integrated circuits (IC), which perform a vast number of tasks and computations.
These items have been adapted and implemented in many devices, and they are becoming increasingly powerful. (Matsui et al., 2019).
Integrated circuit design is a field that
handles the creation of ICs, which make up many different parts of computers (Kaur & Gill, 2016). An integrated circuit (IC),
also known as a microchip, is a miniaturized electronic circuit that contains a large number of interconnected electronic components,
such as transistors, resistors, capacitors, and diodes, on a single semiconductor wafer or chip. The components are etched onto
the chip’s surface using various semiconductor manufacturing techniques, allowing for the integration of complex functionalities
into a small, compact form factor. ICs revolutionized the electronics industry and paved the way for the development of modern
computing systems, consumer electronics, telecommunications devices, and countless other technologies. They are the foundation
of almost all electronic devices, from smartphones and laptops to automotive systems and medical devices. The concept of integrated
circuits was first introduced in the late 1950s by Jack Kilby of Texas Instruments and Robert Noyce of Fairchild Semiconductor.
They independently conceived the idea of integrating multiple electronic components onto a single chip, leading to the creation
of the first practical ICs in the early 1960s. This breakthrough laid the groundwork for the semiconductor revolution and propelled
the electronics industry into a new era of miniaturization and technological advancement (Kilby, J. S., 1976). An example of ICs
includes memory chips, processing chips (CPUs, GPUs), transistors, and resistors, among many others. Typically, these components
collaborate to create intricate computing systems. Alternatively, they may possess fundamental features, providing more elementary
computational options. Their design process is a complex engineering procedure that covers the combination of different parts,
inclusive of semiconductors, in sealed tight containers and terminals to interface with the stored instructions. During the design
process, it is crucial to verify that the circuits will perform their intended functions. Even minor errors in manufacturing could
render them unusable. (Dinu & Ogrutan, 2019).
Artificial Intelligence is the science
and engineering of making intelligent machines, especially intelligent computer programs. It is related to the similar task of
using computers to understand human intelligence, but AI does not have to confine itself to methods that are biologically observable
(John McCarthy, Stanford University, 2007). It is also defined as a field that involves the training of computers to perform tasks
with little to no aid from humans (Kumar et al., 2016). Historical data can be utilized by machines to learn patterns that define
certain processes. With repeated learning, machines can enhance their understanding of these processes and improve upon them. The
ultimate aim of AI is to imitate the problem-solving abilities of humans. Although machines lack the unique ability of the human
brain to develop skills and problem-solving techniques, the AI field is combining the learning abilities of machines with their
efficiency to create computers that can learn and improve in increasingly complex ways. AI has been applied to many fields where
automation is possible, making it a versatile and valuable tool. In recent years, AI has made significant advancements that have
transformed the way we innovate. One of the most notable breakthroughs is the creation of large-scale language models (LLM), a
type of NLP (Natural Language Processing) technology, like GPT-3, 4, that can understand and generate human-like text. This has
led to the development of chatbots, language translation, and content generation applications (Brown,
T. B., Mann, B., 2020). Additionally, deep learning techniques have improved computer vision, resulting in advanced image
and video analysis, leading to significant advancements in facial recognition, object detection, and autonomous vehicles (Dosovitskiy,
A., Beyer, 2020). Reinforcement learning has also seen impressive progress, which has propelled robotics and autonomous
systems to new levels of sophistication and adaptability (Haarnoja, T., Zhou, A., Abbeel, P., &
Levine, S. (2018). Furthermore, AI has revolutionized medical diagnostics and treatment, empowering healthcare professionals
with powerful tools for data analysis and decision-making. AI’s integration has positively impacted various industries, including
finance, marketing, education, and healthcare, driving efficiency, accuracy, and innovation. However, ethical concerns surrounding
AI must be addressed to ensure responsible use, transparency, and the protection of individual privacy and societal well-being.
Reports of the early stages of IC chips
designed by artificially intelligent aided by deep neural network learning have appeared recently (Dinu & Ogrutan, 2019).
Over the last decade, there has been significant growth in the integration of AI technologies within Electronic Design Automation
(EDA) tools. AI has played a pivotal role in enhancing various aspects of EDA, such as design automation, optimization, verification,
and physical design (Todorov, I. and Dabral, S., 2020). Typical AI techniques used in the EDA field include machine learning (ML),
deep learning, genetic algorithms, natural language processing (NLP), and reinforcement learning. Let’s delve into these
techniques further.
ML techniques like supervised learning,
unsupervised learning, and reinforcement learning have been used in EDA for tasks such as design optimization, pattern recognition,
and defect detection (Jiang, L., & Yu, Y., 2021). Deep learning, a subset of ML, has been applied in EDA for image recognition,
layout optimization, and design rule checking (Guo, Y., Liu, S., & Huang, T. (2020). Genetic algorithms are used for optimization
problems, such as floorplanning, placement, and routing (Al-Jawaheri, Y. R., & Sharif, B. S., 2019).
NLP techniques are used in EDA for code
generation, documentation generation, and requirement analysis (Jin, W., Li, Q., Zhang, H., & Yang, J., 2021). Reinforcement
learning has been employed in EDA for automated circuit design and optimization (Azizimazreah, A., & Esmaeilzadeh, H. (2021).
These AI techniques have the potential to improve the EDA domain by enabling faster, more efficient, and intelligent design processes.
In the world of artificial intelligence,
Large Language Models (LLMs) are considered a significant advancement and are currently being utilized in the EDA field for various
tasks. These tasks include automating the verification of electronic designs by generating test cases, which can expedite the verification
process. LLMs are also useful in generating documentation for electronic designs, improving their readability and understandability.
Furthermore, LLMs can suggest improvements to electronic designs, enhancing their performance, reliability, and security.
Although Large Language Models (LLMs)
have impressive capabilities in natural language processing tasks, they do have certain limitations that are important to consider
for the IC design domain. One of the major limitations is the high computational cost for training and deploying large LLMs, which
can be expensive and time-consuming. Additionally, LLMs may struggle with out-of-domain or rare inputs, despite their strong performance
on common tasks. This limitation may lead to numerical errors which is crucial when analyzing electronic circuits. Furthermore,
LLMs heavily rely on the data they are trained on, which can make them less effective when dealing with inputs that are significantly
different from the training data. (Hutchins, W. J., Diehl, V. A., Diersen, M. A., & Wheeler, K. R., 2021). These limitations,
particularly in emerging areas like deep learning and large language models, pose a challenge in implementing rapid advancements
in the IC design field. The rapidly evolving nature of technology suggests that there will be significant progress in the fields
of AI and IC Design in the near future. This will involve new breakthroughs and more intricate processes
Study Problem
The problem lies with microchips design
challenges due to high costs, design complexity, and difficulties in verifying IC circuits, especially in advanced manufacturing
nodes of 7nm and below. Microchip designers face significant challenges that modern technology has yet to overcome. To address
this issue, AI technologies are being explored as a potential solution. (Kovacs et al., 2020). The relentless progress in semiconductor
design and manufacturing has led to a rapid and exponential growth in the complexity, functionality, and performance of integrated
circuits. Traditional design, verification and manufacturing methodologies
struggle to keep pace with the growing demands and to provide efficient programmatic solutions to complete microchip’s project
in a reasonable timelines (IEEE, Vol. 82, No. 5, May 1994). To address these challenges, the integration of Artificial Intelligence
(AI) techniques has emerged as a transformative solution.
The verification process is one of the
most challenging aspects of IC design, as it requires considerable time and resources (Dinu & Ogrutan, 2019). There is a growing
demand for machines with deep learning AI capabilities to aid in IC design and verification from the outset. Once engineers have
designed the circuits and their intended functions, it becomes difficult for machines to confirm their performance post-manufacturing.
The list of items that need to be checked is extensive, including working power ranges, transistor properties, and temperature
ranges. (Shoniker et al., 2017). Engineers can assess the performance of ICs and deduce whether they work or not (Miranda, 2020).
However, the widespread applications of these circuits mean that each performs unique functions. An adaptable verification process
is hard to perform, especially when automation is needed. The changing environmental conditions further complicate the operating
efficiency of these circuits. With the combination of technologies still limited, the study aims to reveal the shortcomings and
why it has yet to be widely adopted.
The reasoning behind this is that the
performance and quality of vital computer components can be enhanced with the appropriate use of AI methods. The self-learning
nature of deep learning AI points towards a more advanced manufacturing process beyond current levels. The revelations will help
the practitioners in the IC design field by ensuring a more accurate verification process with possibly fewer errors and faster.
Despite the use of AI techniques for over
a decade, there is still a pressing need to improve the design of IC for custom tasks. It appears that the adoption of AI technologies
in EDA design tools is progressing at a slower pace than expected (Semiconductor Engineering -AI Adoption Slow for Design Tools).
The growing complexity of chip design highlights the need for new and improved automation processes to achieve optimal results.
However, current technology still requires enhancements to enhance automation. Additionally, there is a gap in finding ways to
reduce the costs associated with chip design, as current methods are expensive. To address this issue, research should focus on
identifying more cost-effective alternatives. Furthermore, there is a need to improve the integration of technologies such as machine
learning and artificial intelligence, which represents a third gap. Therefore, it is crucial to conduct research to develop modern
solutions that can close this gap.
Study Purpose
The purpose of this qualitative exploratory
study was to investigate the gap between the use of AI in technology and its integration into IC design flow. A recurring theme
in numerous previous cases is using qualitative-based research approaches. These approaches are backed up by the need for the area
of study to provide meaningful contributions to computing in terms of performance and better designs (Obermayer & Tóth,
2021). The nature of technology is mainly focused on improving devices for managing the ever-increasing demands of processing.
This condition has prompted computer research to focus on their inner workings translating to support by qualitative methodologies.
Further, an exploratory design was deemed appropriate for this study because it matched up the need to investigate existing approaches
at the time to solutions and look for possible improved ones.
The population of the study was the experts
connected to the design of computer chips through modern approaches. This population covered the practitioners in the computing
fields who partook in creating better-performing ICs. The choice participants were individuals who dealt directly or indirectly
with the designing of ICs, practitioners in the AI and ML fields, and possible researchers who were concerned with the progress
of AI within the IC design arena. The research had a sample size of eight participants, chosen through purposive and convenience
sampling methods. This is a non-probabilistic sampling approach that utilizes participants who best fit the study (Indrayadi, 2020).
The reason for choosing this approach was that the area of study is quite narrow and requires a high set of specialized skills.
This means that the participants had knowledge of the fields in which the topic sought to investigate. Recruiting then occurred
through the utilization of a professional network. Participants needed initial negotiations outlining the purpose of the research
and the objectives aimed to be achieved. As such, voluntary consent was utilized before continuing any questions or activities
posed to these participants. The data was collected through a questionnaire distributed to the participants online. This questionnaire
covered both closed and open-ended questions to gather a wide range of knowledge from the participants. The interpretation of the
data collected from the participants occurred through the Microsoft Excel tool to identify patterns and themes. The findings were
used to compare with the results of secondary data from journals regarding the situation.
The need to create more complex circuits
is ever-rising, with the experts having a hard time keeping up with these needs (Marinova & Bitri, 2021). Gathering as much
information as possible while keeping to the topic and conducting a study to interpret different viewpoints of IC design helps
clear up the complexity. With the extracted knowledge, the study findings produce a set of recommendations and possibilities that
could improve the verification process in ICs design. Further, the knowledge from the study expands on the existing understanding
of AI and IC design. As the use of AI in IC design is still improving, the study expands the possible solutions to an existing
problem. Further, careful research revealed whether existing studies in the areas showed any signs of bias or insufficiency. The
identified literature gaps in the study form a basis for possible future study cases while identifying what needs to be done for
an AI-led area.
Research Question
The
research question aims to investigate how AI can work together with IC design to tackle the challenging problems faced by modern
IC design processes. To address the disconnect between these two areas, bridging the gap is crucial. This obstacle poses a significant
challenge for IC design, and the research aims to explore viable solutions. The objective is to significantly enhance the design
flow of integrated circuits, leading to improved performance and power efficiency in computers and electronics.
Q
How can AI be implemented within IC design,
and if so, what are the options?
Conceptual Framework
Figure 1 represents the study’s
conceptual framework. The key focus of the study centers on AI and ICs. These are the top-level areas the study seeks to improve
by finding existing problems and suggesting solutions. AI divides into subsections where each segment represents a contribution
to the study area. Since AI has received much focus and is in the process of widespread implementation, it still faces problems
associated with its usage (Khakurel et al., 2018). These problems form a key part of the study as they help outline the limitations
the study faces. The history of AI is also crucial in the study as it builds the picture of the issues associated with AI. An additional
part on the implementation of AI in the computing industry helps reveal the possibilities of its usage in ICs. AI is also closely
related to ML, and the inclusion of the latter is critical to this study.
The IC sector covers the processes involved
in designing and creating microchips. The IC topic boils down to the design area, which is the main focus of this study. The IC
design process is the main area that has so far introduced limitations in advances in computing (Stopjakova et al., 2018). This
area is further subdivided into history and challenges faced throughout the design process. Understanding the process is essential
for pointing out the possible improvements and fixes relevant to the study.
Throughout the years, various AI/ML technologies
have been incorporated into IC design tools to enhance the design process, improve efficiency, and optimize different aspects of
the design flow (Liu, Q., Jiang, 2019). Some of the prominent AI/ML technologies used in IC design tools include machine learning
algorithms that optimize design parameters and performance metrics such as power consumption, speed, area, and reliability, resulting
in better design outcomes (Mohanty, S., Chakrabarti, S., & Roy, K. (2014). Predictive analytics are also utilized to predict
potential design issues or bugs early in the design cycle, helping designers identify and solve problems before they become critical.
Design Rule Checking (DRC) ML algorithms are used for efficient and accurate DRC, ensuring designs comply with the required manufacturing
rules and reducing the risk of fabrication issues. ML-Based automated layout generation automatically generates IC layout designs
based on design constraints and specifications, speeding up the design process and reducing human effort. AI/ML methodologies are
utilized for high-level synthesis and optimization of register-transfer level (RTL) designs, resulting in better performance and
reduced power consumption.
Physical Design Automation AI/ML techniques
are used in place and route algorithms to optimize chip layouts, improve signal integrity, and reduce design cycle time. One of
the most important topics is timing analysis and closure ML algorithms to assist in timing analysis and closure by predicting critical
paths and suggesting design changes to meet timing constraints. Another important aspect is silicon yield prediction AI/ML models
to predict chip yield and identify potential manufacturing defects, enabling designers to make informed decisions during the design
phase. In the mixed signal domain, Analog and RF Circuit Design AI/ML technologies have been integrated into analog and RF circuit
design tools to optimize transistor sizing, improve matching, and enhance overall performance.
The use of AI/ML technologies in photonic
IC design tools is also becoming more prevalent, providing numerous benefits throughout the design process. Inverse Design and
Optimization AI/ML algorithms are among the technologies that have been integrated into these tools. They are capable of performing
inverse design tasks, where specific optical properties are identified for a photonic IC, and the AI model generates the corresponding
structure or layout. This allows for efficient optimization of complex photonic devices with the desired functionalities. Device
Simulation and Modeling AI/ML algorithms are also used to simulate and model the behavior of photonic devices, such as waveguides,
couplers, and resonators. ML models can predict device performance, including transmission characteristics and resonant frequencies,
without the need for full numerical simulations, which saves computational time. Material and Process Optimization ML techniques
are used to optimize material properties and fabrication processes for photonic ICs. Photonic Circuit Synthesis AI/ML algorithms
synthesize photonic circuits by automatically selecting and connecting photonic components to achieve specific functionalities.
This speeds up the design process and enables the exploration of complex photonic circuits. Additionally, AI/ML technologies can
be used for process control and monitoring during fabrication to ensure high-quality and consistent photonic ICs (V. Verma, N.
Sherwood-Droz, P. Dong, 2020). It’s important to note that the AI/ML field in photonic IC design is rapidly progressing,
with ongoing research being conducted to incorporate AI techniques into different stages of the design process. With technology
advancements, AI/ML is anticipated to have a more substantial impact in facilitating quicker, more efficient, and inventive photonic
IC design.
The final section involves the combination
of the knowledge gathered in the IC design, AI, and ML. The areas combined include the general knowledge of AI and ML, the changes
experienced in AI, and the changes present in IC design. This knowledge is the main highlight of what it takes for customized chips
with AI capabilities to be created. The knowledge converges to the possibilities AI and ICs can have and makes up the final core
contribution of the study. The study obtains inferences from these topics, collects the problems, and seeks relevant solutions.
Figure 1
Conceptual Framework.
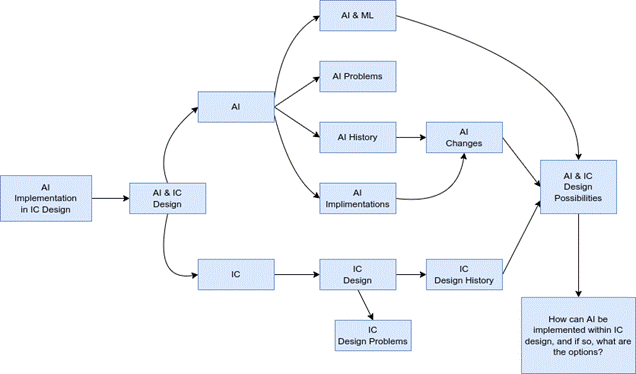
Significance of the Study
The literature review in this study provided
a key overview of the fields of AI and IC design. A majority of the identified literature focuses on innovating methods where one
area helps the other (Chen et al., 2022) and (Moness et al., 2022). However, the study provided a necessary look into the problems
identified so far in general and the solutions so far or the gaps existing. The study is key in contributing to research as the
identified issues are set to be handled and integrated into computing design for better results. The study also contributed to
the technological field and academic area by helping reveal how various methods can be effective in the field of building better
computing chips and the revelation of more solutions areas for research. It is significant that modern research keeps up with technology
in providing meaningful conclusions.
Researcher Positionality and Reflexivity
This research topic is an interesting
area in my related field, which covers the technology behind computers. The motivation to get involved in circuit design and the
inner workings of computers has so far grown from the early tinkering with devices of all sorts. Choosing to focus on AI and ICs
design is also set to expand my understanding of machines while meaningfully contributing to the field. The research is interesting
and shows that the combination of the two areas will soon lead to interesting innovations. The position as an enthusiast and practitioner
in the technology field is set to contribute to the knowledge of AI utilization at the core of chip design and manufacturing. This
understanding is set to fuel the need to finish up the research and contribute to technology in general with no intended bias.
Use of reflection and sound study methods will be employed to reduce the potential for bias.
Delimitations and Limitations
Delimitations represent the extent of
the aim and questions posed by the research as highlighted by the researcher (Theofanidis & Fountouki, 2018). One of the key
delimitations of this study is the exclusion of covering the cutting-edge discoveries and technologies in the field of circuit
design in the literature review. The reason for the exclusion was brought by the need to focus on an approach that identifies the
problems caused by manual processes. The unverified and cutting-edge technologies are limited in substance as such changes take
time to implement and integrate into the existing designs. The research was also set to only focus on the fields of AI and ICs
design. Any topics that deviate from the two were only briefly touched upon if they fit within the research context. The necessary
skillset needed for the participants was in computer science with specializations in circuits design or AI. The study expanded
geographical limitations and included online-based surveys for participants from any part of the world.
Limitations in the study are the points
that are weak and beyond the control of the researcher (Wells-Cornwall, 2021). While theoretical understanding is necessary before
practical applications, this approach is set to carry over weaknesses experienced in the existing studies (Shi, 2018) and (Stopjakova
et al., 2018). The limitations include circuits being prone to sensitivity to temperature, small available spaces, and noise in
the circuits. Other studies pointed to the need for more focus on the practical applications of artificial intelligence with a
thorough exploration of existing solutions. The result of such an approach was a limited understanding of the exact contributions
these studies provide to the solutions. The need for an adequate look at history while being innovative, therefore, was large.
This study was limited to obtaining data from only a small sample size, although this is appropriate for the qualitative nature
of the exploration needed.
Definition of Terms
The terms that follow cover the major
focus areas of the study and are necessary to get the complete picture of how they relate to the research. The terms focus on the
technical aspects of IC design and computing.
AI
Artificial
Intelligence (AI) is defined as applying advanced analysis and logic-based techniques, including machine learning (ML), to interpret
events, support and automate decisions, and take actions. The definition provided aligns with the present and developing state
of AI technologies and capabilities. It recognizes that AI often involves probabilistic analysis, which combines probability and
logic to assign a value to uncertainty (Gartner Glossary).
Circuit Verification
Circuit verification is a process of identifying
how circuits correctly perform their designated functions and whether they produce any errors (Manolache et al., 2022).
Integrated Circuits
Integrated circuits are electronic components
made of semiconductors containing tiny transistors, diodes, and capacitors used to process information (O’Regan, 2018).
Machine Learning
ML is a subset of AI field, focusing on
the approaches and processes that allow machines to acquire knowledge and use data to improve their tasks (Alexopoulos et al.,
2019).
Robotics
Robotics is a branch of engineering that
covers the creation and use of programmable machines (Moradi et al., 2019).
Chapter Summary
This introduction chapter has outlined
the initial information of the dissertation and what areas it covers. To recap, the dissertation focuses on the implementation
of AI in the design of ICs. These areas are key to more efficient machines and ones that have been designed with more complexity.
The study opportunity was to reveal the shortcomings so far and how they have been handled so far. This revelation identified the
gaps and issues that need addressing in the future implementation of IC design. The research question focused on revealing the
possibility that AI could be utilized to enhance the design process of ICs. The study was significant in contributing to technology,
revealing more topic areas for future studies, and expanding the knowledge of computing. This study only focused on the above-mentioned
topic areas and involved a theoretical approach to accomplish its goals. A deeper dive into AI, ICs, and their design was outlined
in the coming literature review chapter.
Chapter 2: Review
of the Literature
The problem lies with the high costs of
designing chips, difficulties in the verification of IC circuits due to the complexity of the process, and limitations in the automation
of the verification process, which modern technology needs improving on and intends be handled by AI (Kovacs et al., 2020). The
purpose of the qualitative exploratory research was to explore the disconnection between AI applications and IC design. The conceptual
framework of the study centers on the implementation of AI in ICs design, which is a lucrative area that is changing modern computing
(Yantır et al., 2022).
This literature review chapter is an important
section of the dissertation as it reveals the existing material and knowledge relating to the areas of study. The material utilized
in this section is peer-reviewed papers, conference papers, and any additional material that is relevant and verifiable. The chapter
is organized around AI and ICs with additional topics which are derived from the areas, as depicted in the conceptual framework
for this study (Figure 1). Further literature is provided on the devices and applications in AI and the design process of chips,
and how it has changed over time. Additional material is presented on AI and IC design, ML and IC design, applications of AI in
IC design, and the progress so far on AI and ICs design. The chapter also covers the automation of processes in ICs design, the
verification of ICs, hardware verification processes in combination with AI and more. The last sections of the literature material
cover the trends occurring in IC designs and a small coverage of detecting issues in ICs. An additional section is present on the
gaps identified in the literature and why they are important to this dissertation. The chapter ends with a conclusion and summary.
Literature Search Strategies
The literature search was conducted through
a combination of the ProQuest Dissertation and Theses database combined with a search on the popular research search engine Google
Scholar. The chosen articles are related to AI and IC design. The paper choice comes through a brief overview of each article and
seeing the knowledge it contributes.
The keywords used in the search for the
documents include artificial intelligence, integrated circuits, integrated circuits design, implementation of AI in IC design,
the progress of AI, machine learning, machine learning models in IC design, errors in IC design, limitations of AI in IC design,
and automatic IC design. These terms are created through frequent updating of the search process and tuning what brings up the
most relevant results. The next sections explore various materials covering the target topics.
Artificial Intelligence (AI)
AI is a critical part of the modern world
with its contribution to computing (Berente et al., 2021). Technology has so far altered our approach to problems, as humans are
starting to solve problems previously considered unsolvable. The contribution of AI has also so far changed the pace of computing
to bring about so many changes than the previous computing contributions combined (Li et al., 2019). The current research is geared
in a direction seeking to improve lives and achieve a deeper understanding of various topic areas. The possibilities are endless
in terms of the implementation of AI, but the major hurdle is towards overcoming current challenges (Berente et al., 2021). These
technological challenges are also part of the issues to face with other areas covering security, ethical considerations, data handling,
rights, social implications and more. The issues being brought up are a necessary step and show the progress so far achieved in
integrating AI (Berente et al., 2021). Such a massive advance in steps means that AI is single-handedly changing so many areas
of life currently and is expected to contribute more. AI is indeed at the frontier of modern achievements.
These advanced changes in computing can
be attributed to the ever-improving changes in the processing capacity of chips (Lu et al., 2018). Performance in computing chips
has been steadily increasing each year, leading to better capabilities of each generation of computers. Coupled with the improvements,
the inclusion of big data in processing is also adding to the capabilities of AI. The changes, therefore, are synonymous, and the
large capabilities can be attributed to these iterative additions. Liu and Chakrabarty (2021) also point out the eventual need
for AI to operate at similar capacities and capabilities to the human brain, an ambitious move set to reveal key details in computing
and in solving major problems that have been available throughout human existence.
Datasets powering technology and AI keep
getting larger as access keeps on getting easier (Wu et al., 2021). These changes mean that AI is expanding at a fast rate in all
industries and areas. Significant challenges, as illustrated by Wu et al. (2021), show that the implementation is posing limitations
with the current capabilities humans possess. The growth of data is occurring at a larger pace than the progress in keeping up
with its processing, whether in storage, processing, or its utilization in decision making. Hampton (2020) point out the need to
be innovative and quickly come up with the necessary tools to balance out the growth rates.
Different-sized machines are also claiming
a stake in their contribution to AI (Srinivasulu & Ravariu, 2020). From large equipment capable of spanning buildings to tiny
devices that might be invisible to the naked eye, AI is changing all areas. Nanotechnological devices and tools are also seeking
to adopt AI and enhance the future of computing and gadgets. These devices, as reported by Alrubei et al. (2022), might be more
efficient in terms of costs and integration in different areas. The downsizing of the tools also means that their spreading to
more capabilities will not be limited by size, portability, or restrictions of current machines.
The sentiment on AI operating at a scale
to the brain has prompted issues on its replacement of human workers (Hassani et al., 2020). These worries come from the ever-improving
robots powered by autonomous machines, which work at better rates and efficiency and without the need to take breaks. Davenport
and Ronanki (2018) showcase the possibility that such a case creates panic among everyone. However, their study shows that most
jobs are not about to be easily automated and push people out of their positions. This means that AI is not yet efficient enough
to replace human workers.
A review of the current literature and
direction of AI and robotics by (Li et al., 2019) shows a direction toward mimicking naturally occurring items like humans and
animals. These machines are built to learn about the movements of the items and learn from the items that are not working. The
eventual progress leads to a set of machines that can keep on improving to perfection. The AI models used to train and power these
robots are improving each passing year (Alam, 2021). The number of laboratories, institutions, and private companies rushing to
achieve such autonomy is quite high. These moves are set to improve the performance capable of modern robotics and computing machines.
The approaches in training the machines are split into top-down solutions where the larger problem is subdivided into smaller sections
and a bottom-up approach concentrating on the combination of smaller solutions to solve more complex ones.
Generative Pretrained Transformers (GPT)
is an advanced AI technology that has revolutionized the field of natural language processing. These models are built on the Transformer
architecture and are pre-trained on vast datasets to learn language patterns and structures. GPT models excel in generating human-like
text based on given input or context.
One of the most notable GPT models is
GPT-3, developed by OpenAI. It is one of the largest language models to date, with 175 billion parameters. GPT-3 has proven to
be highly effective in tasks such as text generation, translation, question-answering, and language understanding (Forbs –
Innovations).
GPT-4 was released on March 14, 2023,
and is currently available in limited form through the premium chatbot product, ChatGPT Plus. Access to the GPT-4 based version
of OpenAI’s API is provided through a waitlist. The number of parameters in GPT-4 is unconfirmed, with some speculating that
OpenAI has used around 100 trillion parameters, while others claim it has 1.76 trillion parameters (Wikipedia - GPT 4).
The pre-training process of GPT involves
training the model on vast amounts of text data from the internet, enabling it to learn grammar, syntax, and semantics. This pre-training
is followed by fine-tuning on specific downstream tasks to adapt the model for more specific applications.
GPT and other generative models have various
applications in natural language processing, creative writing, chatbots, language translation, content generation, and even code
generation. These models are pushing the boundaries of what AI can achieve in language understanding and generation tasks.
AI has an ultimate goal to achieve superintelligence
(Müller & Bostrom, 2016). The current advances are still in their early form, yet to get to general intelligence. The
next massive step in AI is expected to reach superintelligence. This state is possible where the machines will have capabilities
in processing information beyond the point and comprehension of humans (H. Lee, 2022). The intelligence is also expected to span
several areas, and the machines will sail through without any issues.
It is expected that AI is soon set to
infiltrate every part of human lives and interactions (Agrawal et al., 2017). From the internet, social media, and more, AI is
already powering most of the behind-the-scenes choice of content for everyone. This slow creep into everyday tasks means that AI
will go deeper than personal lives and alter our interaction with it (Sparrow & Hatherley, 2020). It is set to be part of human
lives and involved in making decisions, interacting on our behalf, and more. The capabilities of AI have since been elaborated
in multiple types of research and show an infiltration beyond current technology.
AI Devices
The technology utilizing AI is widespread
across different industries such as robotics, transportation, health, and sensing (Srinivasulu & Ravariu, 2020). This observation
shows that devices are getting better at processing information and will soon be autonomous. Nano devices are also included in
the utilization of AI, but their progress is slow following limitations in circuit design (Srinivasulu & Ravariu, 2020). The
teaching region is also experiencing the utilization of AI (Bailey, 2019). This area is crucial in providing learners with the
correct basic skills to experience an ever-improving cycle associated with AI. AI is also set to personalize the learning experience
through such adaptation. The major effort responsibilities, therefore, lie with the semiconductor manufacturers who need to create
devices that match the software demand of AI (Batra et al., 2019). The extent of devices expected to handle AI and integrate it
into everyday lives, therefore, keeps growing as the capabilities and manufacturing capabilities expand (Batra et al., 2019).
Integrated Circuits
Integrated circuits have altered the entire
electronics industry by introducing their unique approach to devices (O’Regan, 2018). Their evolution from large sizes containing
a few transistors to tiny items with millions of transistors shows major progress in the manufacturing and computing world. The
cost of constructing these circuits also reduced dramatically, leading to their inclusion in almost all electronic devices currently
(O’Regan, 2018). Hao et al. (2021) highlighted that the improving circuits are ever getting smaller and more powerful following
Moore’s law. Moore’s law states that the transistor density in chips doubles every 2 years (Xiu, 2019). Modern ICs
make up most of the processing technology and have changed through different generations to be extremely complex devices.
The processing of the chips takes different
approaches, which involves the chemical processing of the semi-conducting material to allow the processing needed (Ilagan et al.,
2020). Algorithmic processes have been invented to create complex circuits at a tiny scale, with some having a few nanometers separation.
This scale indicates the tiny margins of error that the manufacturers work with and the many possibilities of errors occurring
(Ilagan et al., 2020). Some technologies, such as the nanoimprint lithography technology, are variations towards getting the most
precision in the devices (Sreenivasan, 2017). The approaches seek to go further down and handle the semiconductors at a molecular
level, which is a long stretch considering the initially ICs developed after their discovery.
Chip Design
The increase in complexity of chip design
has been on a steady rise with the development seeking shorter design and development times (Obilisetty, 2018). Chip design stands
as one of the most complicated processes in creating computers or electronic items (Richelli, 2021). This complexity arises from
the large number of variables needed to be considered when handling the chips to make them reliable, resilient, and more. The need
for chips that use low voltage is also rising, further complicating the design process (Richelli, 2021). Some relief is pointed
out by Obilisetty (2018) on data and intelligence-based approaches being applicable to ease the processes of designing and creating
chips. Other approaches are seeking to create designs that are aware of the energy they use and can route the processing depending
on conditions (Chaudhary et al., 2020). The approaches are also raking up the performance and speeds of the chips while trying
to design them for operating in multi-chip environments.
An overview of chip designs from their
invention in the 1960s shows a change from tens of micrometers to recent micro distances of a few nanometers between the circuits
(Liang, 2021). The designs and manufacturing are a tight race for the most efficient and powerful designs that are quickly implemented
into everyday items. The inclusion of photonic ICs also expands the capabilities of modern chips in terms of applicability (Kish
et al., 2018). This further expands the possibilities of chip designs to exclusive functions such as optical-based technology and
where the processing is more efficient in their applications.
The current general direction is towards
the best performance, high security, very low power consumption, and well-connected ICs (Kulkarni, 2020). Modern designs are also
evolving to customize chip design and manufacturing for each industry for a particular functionality. Kulkarni (2020) is keen to
highlight these changes and state the declining application of analog designs in favor of automated ones.
Artificial Intelligence and Integrated
Circuits Design
AI is creeping into many different areas,
including IC design, where special chips are being invented for its execution (Monroe, 2018). The computational needs and power
consumption of AI mean that the traditional ICs are not well equipped to handle it. The result is the creation of hardware that
targets ML and AI needs (Monroe, 2018). The design process of ICs for the implementation in AI means that the chips need to be
verifiable in terms of mathematical specifications (Seshia et al., 2022). Seshia et al. (2022) also outline that the specifications
of AI chips are at a different level from typical ones. AI-based chips need to operate in a distributed manner far from the central-based
designs of typical computers (Shastri et al., 2021). These diverse chips are set to mimic the diversity of brain cells which operate
in unison despite being stand-alone in their actions.
There exist some approaches that focus
on applying AI and ML to Integrated circuits design. Approaches such as the Very-large-scale integration (VLSI) are used in designing
and testing circuits and use AI to perform automation and repetitive tasks (Amuru et al., 2023). The scale of IC design has also
increased to the point where human engineers cannot design circuits without the help of machines.
Some special types of IC designs need
to integrate functionalities such as wireless, sensors, and more (Fayazi et al., 2021). The need for these complex chips for multi-purpose
use means that their design, verification, and consumption of power need to match the changing times. The chips also need to be
secure as the cases of cyber-attacks, and the need for data protection continue to rise (Xu & Zhang, 2020). Xu and Zhang (2020),
in this case, are focusing the design process on handling the fast-growing insecurity cases on computer-based machines. The propositions
are towards designing the chips from the ground up with tight security and preparing for a tough environment (Xu & Zhang, 2020).
Machine Learning and Integrated Circuits Design
A revisit of Moore’s law points
to an exponential increase in chip power and performance with every passing year (Khailany & Dally, 2020). Modern approaches
are being utilized to accelerate the performance of chip design that covers different areas such as their verification, testing,
timing, and more (Khailany & Dally, 2020). ML approaches are currently being utilized in this modern design to accommodate
the complexity and data requirements (Li & Franzon, 2016). All manufacturers are currently rushing to use the latest approaches
in ML to increase the manufacturing process and ensure its eventual success. ML has since proven to be excellent in improving these
processes (Li & Franzon, 2016). The pressure in competition on the ICs design pushed early designers to seek alternative approaches
to the processes (Kahng, 2018). These processes have since been replaced by predictive models that look for any issues in the pipeline
and estimate how well they can be solved (Kahng, 2018).
There is an ever-increasing need to design
chips that are cheaper in terms of development, integration, maintenance and long-term use (Saito et al., 2020). The researchers
point out that such an achievement requires complex expertise in creating the best chips and ensuring that they can last the intended
lifetimes (Saito et al., 2020). The testing process is highlighted as the most important part of developing IC chips (Amrouch et
al., 2021). These issues on current ICs point to utilizing ML for a deeper analysis that the analog-based processes cannot comprehend
(Amrouch et al., 2021).
The automation of the design of analog
circuits through ML and artificial neural networks (ANN) is also an ideal approach (Devi et al., 2021). The ANN models provide
a self-improving approach different from the typical ML algorithms and with better expectations in the outcome. However, all the
design processes boil down to the reliability of every single chip that the ML approaches are trying to handle (Jiahe, 2020). The
general agreement seems to center on applying advanced ML models to seek out problems and suggest the best approaches to make them
affordable for the developers and their applications (Jiahe, 2020). Open sourcing the design processes or the optimization options
is also proving to be a desirable approach (Zezin, 2022). The growing complexity of Integrated Circuits design means that the variables
to consider are ever-increasing, leading to the need to include as much expertise as possible (Zezin, 2022).
The applications of ML in IC testing are
also being steadily expanded to overcome the existing problems (Stratigopoulos, 2018). With the availability of large datasets
being integrated into ML processes, detecting any issue in design and manufacturing is proving much easier (Stratigopoulos, 2018).
These changes have been forced by the rapid changes in technological advancements that have since been hard to keep up with (Afacan
et al., 2021). The changes can be attributed to the need for accurate responses and the challenge of keeping up with fast-paced
competitors. Automation tools for analog circuits and chips are increasing at a high rate and proof of the demand (Afacan et al.,
2021). The traditional approaches have since been declared inefficient and time-consuming, which do not add up to the expected
results (Mina et al., 2022). Manufacturers are currently relying on the input from ML algorithms to improve efficiency and point
out the areas needing improvement or replacement (Mina et al., 2022).
A different approach focuses on the design
and development of chips designated for ML (Sze et al., 2017). This approach aims to identify the changes in trends and create
the exact chips that can easily be applied for ML, robotics, and the Internet of Things (Sze et al., 2017). The identified opportunities
for ML in IC design revolve on pushing the limits of the problems presented by electronic designs (Wang & Luo, 2019). Such
problems are frequent in a world geared towards the eventual overcoming of chip design challenges and integration and use of multi-chip
machines (Wang & Luo, 2019). ML is indeed a recurring concept in designing modern chips and ensuring their affordable nature
and high performance.
AI Applications in IC
It is impossible to separate AI and ICs
(He, 2021). This combination comes from the dependence of AI to run on ICs at the core. The performance of intelligence and learning
ability comes from how efficiently the ICs have been designed. The growing complexity has seen the application of AI in business
processes (Pheng & David, 2022). The IC manufacturing industry has also seen the use of AI in recent times, where the demand
for the best performances keeps increasing. The timely need to deliver the chips at the correct time is also ever-pushing.
Circuit logic simulation is a critical
area where AI is set to revolutionize (Lin, 2021). The application also extends to the optimization of 3-D ICs and the systems
they power (Park et al., 2017). These areas are key in determining the future of AI, as they mean the best outcome for computer
chips. In the logic simulation, AI applies to checking the different combinations possible and the best way to improve them (Lin,
2021). These improvements lead to the scrutiny and handling of any errors that might arise in the processing of information. The
3-D integration processes contribute to more dense systems, which eventually bumps up the performance.
Another approach is in distributed AI,
where special circuits are being built to process data on wireless networks of sensors (Chêne et al., 2022). This area is
expected to improve on the current wireless networks and more adaptive processing using AI. The possible challenges to solve are
security, energy efficiency and more.
Progress on AI and IC Design
The progress AI has contributed to IC
Design is massive so far. AI is bringing about the optimal design of modern chips by easing the process (Beres et al., 2015). The
example of AI being used for passive damping to handle the issues brought about by the resonance coming from high-order filters
shows progress. Further, AI is also used in the testing processes where the traditional methods are costly and time-consuming (Liu
& Chakrabarty, 2021). The application of AI and ML in these tests ensures that the optimal solution is picked and thus reduces
the entire cost of testing these chips (Liu & Chakrabarty, 2021).
Processing in memory is also a new type
of approach which are turning out to need a new iteration of IC chips (Kim et al., 2022). The AI and ML approaches are being utilized
to handle the high processing functions and processes needing large amounts of data. The trends point toward better-designed chips
at the bank level that are much more energy efficient than the traditional ones (Shanbhag & Roy, 2022). The mapping of the
in-memory computing processes in the design processes is proving a complex undertaking that ML is helping on. The advantages are
also evident in the scaling process of the technology in terms of energy efficiency and handling the high-density circuits (Shanbhag
& Roy, 2022).
Miceli et al. (2022) mentions the application
of AI to design chips utilized in deep space astronomy. Such actions mean the transfer of data and processing is high and needs
high-performance IC chips. The features being introduced by AI are shown to be better at scaling the computing processes on specially
made chips (Miceli et al., 2022). An additional area lies in photonics, where neural networks are utilized to design the necessary
chips (Alagappan et al., 2022). It’s important to note that the field of AI/ML in photonic IC design is rapidly evolving,
and more research is being conducted to explore and integrate AI techniques in various aspects of the design process. As technology
advances, AI/ML is expected to play a more significant role in enabling faster, more efficient, and innovative photonic IC design.
Automating IC Design
The process of automating the design process
of ICs is a developing concept aiming for the accomplishment of more complex approaches (Huang et al., 2021). This automation is
growing fast following the training of ML models, which are accumulating knowledge for the best outcome. The traditional approaches
are set to be overcome by the automation ones solely from the knowledge accumulation of the design process (Huang et al., 2021).
Analog and physical design of circuits
had dominated their creation for a long time (Youssef et al., 2020). The suggestion of using frameworks to utilize look-up tables
to compute the circuits is gaining traction. This approach is seen as more accurate and faster when it comes to simulating the
design process (Youssef et al., 2020). The process is also said to enhance the decision-making process and innovative options for
IC design processes. The analog design process is also a process that relies heavily on the experience to explore the tweaking
options of the simulation process for better circuits (Youssef et al., 2020). The major issue in this process, termed a bottleneck,
is this part costs more in terms of resources and time necessary. Electronic Design Automation (EDA) tools have since emerged to
replace traditional approaches (Nardi et al., 2019). For autonomous driving applications, the design of extra complex chips is
needed at a high pace (Nardi et al., 2019). The automation potential is steadily increasing as better implementations and regulations
are devised (Nardi et al., 2019).
The complexity of IC designs in the matter
of cost is also termed as a large barrier in designing circuits (Kahng, 2018). It is termed more costly to scale down circuits
for more dense circuits, which improves the performance and allows for better processing. The opportunities for automation and
better designs are wide and are set to alter the entire computing industry (Kahng, 2018). It is also clear that automation balances
the process by better inclusion of ML, better contribution from designers, and deeper integration of more design tools. Demand
in the ICs for improved computing options is also significantly high (Gubbi et al., 2022). EDA is currently termed as a necessary
enabler of IC designs and the semiconductor industry in general. The learning approaches currently available have potential improvements
by applying ML methods as their source of analysis. The accumulation of data for the learning process is also expanding as designers
are exploring better alternatives (Gubbi et al., 2022). These improvements are experimented across all types of ML learning, such
as supervised, unsupervised, semi-supervised, and reinforcement learning. Casting a wide net on the possibilities is viewed as
a better option in revealing key contributions to better ICs (Gubbi et al., 2022). From getting rid of unnecessary designs and
optimizing the best existing processes to exploring innovative ways for physical designs, the opportunities for automation are
endless.
The feasibility of automation options
is definitive, given the situation in the physical design of circuits. A ML approach by (Islam, 2022) showed a reduction in 98%
of violations associated with IC design. The implications of automation’s contribution to computing are huge and anticipated
as the expected next move (Islam, 2022). A suggestion by Gubbi et al. (2022) combines the greedy searching algorithm with an ensemble
approach to get better performance for detecting issues in circuit design. The result is better efficacy of the algorithms and
which is intended to improve general ICs.
The layout of the Integrated circuits
is also an important part to consider in the analog IC design (Scheible & Lienig, 2015). The process of designing analog ICs
also takes time, with automation coming in handy to reduce it significantly (Porrasmaa, 2021). The newer approaches are integrating
approaches that overcome the current constraints and creating designs that are context-aware (Scheible & Lienig, 2015). Tests
are showing the approaches might soon be integrated as the complexity of ICs keeps on getting complex. The reusability of the automation
processes is high, which points to the maximum optimization of integrated designs in the future (Porrasmaa, 2021).
A new approach called the Analog Layout,
Intelligently Generated from Netlists (ALIGN), is set to automate the layout of analog circuits and shows signs of good performance
(Dhar et al., 2020). This approach utilizes algorithms and templates to ease and make the designs much better than the traditional
approaches. Integrating AI in the learning process is so far improving the errors made by other automated processes. The demand
for better hardware is changing the design scene by introducing the need to explore alternatives in the design process (Mina et
al., 2022). The encouraging results from this integrated approach show a better future in IC design (Takai, 2020).
Some developments in the design of ICs
are introducing unforeseen challenges in the automation process (Chu & Ozdal, 2018). The nature of the design process shows
that it might need new ML models to try new approaches.
Circuit Verification
Verifying circuits for errors is an ever-important
part of their design and is getting more complex (Fan et al., 2020). Designers have a tough job of creating the circuits within
tight restrictions but are expected to perform their best. The verification process designers are slowly adopting systematic approaches
to meet these restrictions (Fan et al., 2020). An approach using decomposition workflow has shown to be one way to verify the chips
(Kimura et al., 2020). The approach also introduces a collection of testing bits that contains errors for the verification process
to unearth. The approach shows good performance in the verification with a reduction to days down from months using other processes.
With the reliability of ICs being highly
expected in modern chips, it creates a collection of complexities that are hard to handle (Stempkovskiy et al., 2017). The researchers
here use an approach of searching for faults in the logical circuits aiming to reduce the number of checks. They achieve this by
looking for one of each set of faults without repetitions for similar issues (Stempkovskiy et al., 2017). Advancing technology
is enabling additional verification approaches for mixed function circuits (Martins et al., 2017). The challenge faced by these
researchers is the increased complexity of the multiple functions, which compounds the possible errors. The approach takes a route
to handle mixed-signal verification processes on the IC structures (Martins et al., 2017).
Simatic et al. (2017) focuses on the design
process of self-timed pipelines where the proposed framework uses a mere and split flow to target the circuits. This approach also
performs high-level simulation for the design process, which is key in easing the costs of the verification and tests. An additional
approach to testing ICs through regression testing also shows the promise of high performance (Cieplucha, 2019). The method is
different as it manages the test structures in a dynamic manner which constantly adjusts for performance improvements during the
simulation.
Hardware Verification and AI
The opportunities and possibilities presented
by AI in verifying hardware during the ICs design process are crucial for their utmost performance (Ogrutan & Dinu, 2019).
The time-consuming nature of the hardware verification step has pushed engineers and designers to look for ways to reduce the time.
AI is turning out to be the best option with its capabilities of learning and adapting to new problems (Ogrutan & Dinu, 2019).
Within the hardware verification process, functional verification also takes a significant amount of time (Dinu et al., 2022).
Investments are currently heading towards using AI to reduce this time and make the process much quicker. Generic algorithms are
shown to make it better for functional verification when compared to the traditional approach (Dinu et al., 2022).
Current hardware verification approaches
utilize the pseudo-random generation of numbers to provide the necessary input (Gaur et al., 2019). The approach leads to a problem
of unexpected output, which in turn leads to unintended failures. The researchers propose a ML model which traces the failures
to the related inputs for the debugging process, which is proving better. Additional research and the creation of new system architecture
are also expected to keep improving the verification process (Mittelsteadt, 2021). AI is intended to cover the verification, assess
performance, and improve the security of the chips.
Security concerns on malicious items being
integrated into ICs by un-trustable manufacturers are turning out to be a major concern (Dong et al., 2019). The existing verification
processes are also not capable of detecting these situations, with the option turning out to be AI. The Internet of things is expected
to rely on such AI-Based techniques for quick and accurate verifications.
The scalability of the verification process
is also an issue, as pointed out by (Shiraz & Hasan, 2017). The issue occurs from the complexity of the process, which provides
hurdles in the mass verification of several designs. An approach to utilizing an AI-based library is showing better capabilities.
IC Design Trends
Numerous areas are being integrated or
created for ICs, including big data, AI, the Internet of Things, Blockchain, robots, and more (Chan et al., 2022). These trends
are leading to an explosion in IC designs, and they need their customizations to handle the unique functionalities never experienced
before. Innovators are also working hard for intriguing designs that are best in precision, efficiency, and low on energy (Chan
et al., 2022). Autonomy and control in IC design are at their initial stages, but the innovation process is currently underway
(Li et al., 2022). The design of ICs is also concentrated in a small area of a few manufacturers.
Sustainability in IC design is also an
area to consider, especially for chips that utilize low costs and can be manufactured at low costs (Kline et al., 2017). Some approaches
are using IC fabrication which targets the low energy consumption cases. Another approach is focused on a multidisciplinary approach
which aims to diversify the outcomes (Klinefelter, 2020). The use of digital tools is an expected trend that is set to improve
the general design of the chips. Open sourcing, agile development, and more engineering and computer science expertise are also
slowly making the chip design process better (Klinefelter, 2020). Scaling the design process is also a lucrative area, as it means
more unique chip designs being rolled out in larger numbers (Kahng, 2018). Deep and ML is set to take over the design and verification
process resulting in more efficient processes (Kahng, 2018).
IC Issue Detection
Designing resilient circuits that can
detect and handle errors is a complex but necessary task during the design stage (Lodhi et al., 2019). The testing and verification
process is geared toward running the chip in its worst case and determining its performance. Handling dynamic problems is also
critical to ensure the chips get the correct voltage and data and always process information with high accuracy (Lodhi et al.,
2019). Some processes utilize a patching mechanism that handles the security problems on the chips (Liu et al., 2022). The result
is flexible processes of the design process and ever-improving designs of the chips. The researchers have also revealed that knowledge-based
patching works better than random searching for issues (Liu et al., 2022).
Anomaly detection is also an issue that
Chen (2018) was able to handle through time series forecasting. The prediction models work on the design data produced by the analog
design processes. The approach combines a predictive process that integrates into the issue detection and can help raise the odds
of success (Chen, 2018).
ML processes are also being utilized for
detecting the issues, especially in the more complex chip design processes (Khalid et al., 2020). The models help in detecting
trojans hidden in the hardware by observing the timing processes, power, communication patterns, and current of the chips. The
possibilities are endless of the capabilities the approach provides (Khalid et al., 2020).
Scanning for issues on ICs, such as possible
cyber-attacks, is turning out to be a vital process (Vashistha et al., 2018). Trojans are increasing in their penetration, where
they are being observed at lower levels in circuits. The hardware trojans take attacks to a new level where they are harder to
handle and get rid of. Detecting trojans is currently difficult as there is no universal approach to handling them (Vashistha et
al., 2018). An approach by (Vashistha et al., 2018) attempts to use a technique of using SEM imaging to look for evidence of trojans
in circuits. This approach utilizes ML and algorithms of vision to check changes in the circuits for misbehavior. Hardware trojans
are also being stated as a large problem that can be classified as a war (Bhunia & Tehranipoor, 2018). Their devastation is
higher than other software-based trojan cases, which so far are easy to detect and handle. The research on trojans is increasing
rapidly, gauged by the increasing cases of research targeting them. The workforce needed to handle hardware trojans is reportedly
huge (Bhunia & Tehranipoor, 2018). This difficulty comes from the need for the testing and verification of the many steps involved
in verifying circuits.
Trust issues are common during the creation
of micro-electronics, where the increase in complexity introduces a lot of participants for components and processes necessary
(Hoque et al., 2020). This complexity has led to many participants needing to specialize in crucial components along the chain
of manufacturing. For sensitive applications such as military cases, trust is a crucial component of IC design and manufacturing
(Hoque et al., 2020). The appearance of hardware-based trojans on modern chips is also a testament to the issues faced by their
creation (Vashistha et al., 2021). The entire supply chain of manufacturing ICs has so far been focused on improving methods to
address the threats. Cases are being created where the verification process can be confirmed and checked (Vashistha et al., 2021).
The physical inspection of ICs is possible
and can detect design flaws in circuits (Asadizanjani et al., 2021). Design flaws are based on the layout of the transistors and
their connections. The current testing process is noted to have flaws and introduces issues such as noise in the circuits (Asadizanjani
et al., 2021). The test patterns can be noted as insufficient and hinder the capability of ICs. Validation process through physical
inspections and comparisons with the intended designs. Introducing the ML comparison mentioned previously is one of the ways modernizations
of circuit design is being implemented (Chen et al., 2022). The assurance of hardware verification stands as a bottleneck in the
design of circuits.
An additional approach to detect issues
in IC design is the use of deep network algorithms that learn the problems associated with the processes (Naswali et al., 2019).
Deep neural networks are different types of ML algorithms mimicking the learning process of humans. The novel implementation by
Naswali et al. (2019) shows an improvement to over 90% accuracy in detecting issues. The general reduction in the effort of the
additional confirmation process is 60%. The focus on these deep neural networks is also illustrated by (Sharma et al., 2021) through
the use of image classification. The test shows a reduction in the noise in the image processing of more than 90%, pointing to
a better approach to detecting hardware errors. The increase of around 15% in better detection rate compared to existing approaches
is a testament to the capabilities of neural networks and their contribution to better-ICs design (Sharma et al., 2021).
Gaps in the Literature
The need to improve hardware is pushing
for ICs to be created for specific tasks (Greenstein, 2021). Although there have been significant advancements in AI technology,
the development and production of integrated circuits remain a complex process, particularly as we transition to smaller nanometer
processes. Furthermore, microchips are expected to constantly grow in complexity due to the ongoing advancements in semiconductor
technology and the increasing demand for higher performance and functionality in electronic devices.
As the semiconductor industry follows
Moore’s Law, which predicts that the number of transistors on a microchip will double approximately every two years, the
complexity of microchips continues to increase exponentially. This continuous scaling of transistors enables the integration of
more computing power into smaller and more efficient chips.
Moreover, with the rise of technologies
like artificial intelligence (AI), internet of things (IoT), 5G, and autonomous vehicles, there is a growing need for microchips
that can handle complex tasks and process vast amounts of data quickly and efficiently. This demand drives the development of more
sophisticated microchips with specialized functions and improved performance (Kapoor, A., Lin, R., & Green, W. M. (2019).
There is a gap between two areas that
need to be bridged for machines to effectively assess the situation, receive a requirement list, and efficiently design custom
and synthesized chips. This will reduce the need for vast human efforts and intervention during many time consuming IC design stages.
However, designers must still approve and sign off on the final results generated by automation tools to make sure the desired
outcome is achieved. The chips of the future are also being pushed to be as low-cost as possible without compromising on their
performance (Myny, 2018). This reduction in cost allows for the implementation of affordable computers and allows their mass production
at lower budgets. However, the added complexity makes the design and implementation process quite expensive for the latest design
features to be present. This gap means that there is a need to research alternative options for either reducing the complexity
or automating the process in a way that can be delegated to machines. Having computers designed and offering options can be an
excellent alternative to the existing ones.
The EDA industry has incorporated AI technology
within commercial, IC design, verification, and simulation tools for over a decade. Leading companies like Cadence (cadence.com),
Synopsys (synopsys.com), and Siemens (siemense.com) are implementing advanced AI and ML techniques within their software tools
to address modern ICs’ size, vast amounts of data to analyze, complexity, and other design factors like design rules, thermal
analysis, and reliability. The continuous evolution of AI technology in this field is expected to provide IC designers with further
intelligent productivity enhancement tools.
Synopsys.ai is the first EDA solution
suite to use AI from system architecture to manufacturing, providing AI-driven solutions like digital design space optimization
to achieve power, performance, area targets, and boost productivity. Other EDA vendors are also expanding the use of AI and machine
learning to incorporate multiple tools, providing continuity and access to consistent data at various points in the semiconductor
design flow (Talati, N., & Saha, I. 2020).
Cadence Design Systems has been a leader
in this area for many decades, incorporating AI and machine learning techniques into its EDA tools for analog circuit synthesis,
optimization, and design exploration tasks (Cao, Y., Chen, T., Zhang, X., & Zhang, J. (2020). The Cadence Joint Enterprise
Data and AI (JedAI) Platform can harness the rich lode of EDA data in an open, AI-driven, large-scale data analytics environment,
allowing engineering teams to visualize the data, uncover hidden data trends, and automatically generate design improvement strategies,
leading to improved design performance and engineering productivity. Using advanced AI techniques, Cadence EDA tools are engineered
to produce higher-quality ICs faster than ever. Implementing AI/ML technology enables a significant boost in synthesis, verification,
and simulation arenas.
Mentor, a Siemens Business, EDA tools
use AI for tasks such as floor planning, place and route, and timing optimization, enabling faster design iterations and better
design quality. The Siemens Xcelerator portfolio includes an AI and ML solution uniquely positioned to help companies leverage
AI- and ML-powered EDA tools to deliver differentiated AI- and ML-driven innovations to market faster. The Siemens.ai EDA suite
includes AI-driven solutions such as digital design space optimization to achieve power, performance, and area targets and boost
productivity (Verma, A., & Pedram, M. 2019).
Human skills still play a crucial role
in microchip design and are considered superior to AI in certain aspects, particularly in Analog, MIXED SIGNAL and RF designs.
While AI and machine learning techniques have made significant advancements in the field of microchip design and are being increasingly
integrated into the design process, human expertise remains essential for complex and innovative designs (Wong, K. S., & Mitra,
S. 2019). Particularly Analog chip design involves dealing with continuous signals and complex behaviors, which makes it more challenging
for AI to achieve the same level of expertise as experienced human designers. Analog and RF chip designs often requires deep understanding,
intuition, and creativity to address various challenges, such as noise, parasitic effects, and variability. Human designers possess
years of experience and domain knowledge, allowing them to make informed decisions and design circuits that meet specific performance
requirements (Tajik, A., & Samavati, H. 2019).
An AI can serve as a valuable tool for
improving design productivity by automating time-consuming tasks like layout optimization, placement, and routing. This can significantly
reduce design time and enhance efficiency. However, the expertise of human designers is often required to make critical decisions,
creatively solve problems, and push the boundaries of microchip performance and power efficiency. Designing advanced microchips
involves dealing with complex trade-offs between performance, power consumption, area, and cost. It requires a deep understanding
of circuit design principles, materials, and manufacturing processes. Human designers possess intuition, creativity, and domain
knowledge that enable them to make informed decisions and come up with novel solutions that may not be achievable through automated
AI algorithms alone (Cao, Y., Chen, T., Zhang, X., & Zhang, J. (2020).
These gaps identify the areas of focus
of this study as the progress in AI and IC design is determinant of the emerging technologies in the near future. This study will
focus on looking for the automation capabilities of AI in IC design forming the baseline of the needed changes in technology.
Conclusions
The literature shows that AI is a quickly
growing area with a lot of possibilities and implementation options (Dauvergne, 2021). The integration of AI technology in the
world of IC design has marked significant progress, promising breakthroughs in performance, automation, and efficiency. However,
when it comes to tackling the complexities of advanced nanometer nodes, AI faces challenges related to perception, performance,
and capabilities. The continuous shrinking of technology nodes has enabled the fabrication of advanced nanometer ICs with unprecedented
complexity and performance. The design and optimization of such chips demand advanced methodologies, and AI has emerged as a promising
solution to meet these requirements (Bhardwaj, S., Chakrabarti, C., & Cao, Y. 2020). AI has transformed various stages of IC
design, including layout synthesis, physical design, verification, and optimization. Neural networks, machine learning algorithms,
and reinforcement learning techniques have shown remarkable progress in automating repetitive tasks, exploring design spaces, and
accelerating time-to-market.
With all modern electronic devices being
powered by ICs, it is turning out to be important to concentrate on the efficiency of designing and creating them. The IC design
process is a complex area that AI is currently helping to streamline (Fujita, 2019). It is clear that the increasing complexity
of IC design requires the formation of AI based frameworks and models that help ease the process.
AI technology is driving advancements
in IC design, but there are still challenges in dealing with advanced nanometer nodes ICs related to perception, performance, and
capabilities. As the design and manufacturing of microchips present new challenges based on the laws of physics, the need for faster
and more effective EDA tools is expected to grow. Circuit design is becoming more complex, manufacturing nodes design rules are
getting smaller, and there is a demand for low power. These factors create many opportunities for AI technologies in the IC design
domain (Liu, S., Yin, Y., Yu, M., & Hu, C. 2020).
AI models face challenges in accurately
perceiving the intricacies of designs at advanced nanometer nodes. Factors like process variations, electromigration, and parasitic
effects introduce uncertainties that AI must account for in its predictions. AI-driven tools are currently encountering performance
limitations when dealing with large-scale IC designs, high-dimensional design spaces, and computationally intensive simulations.
Hardware accelerators and distributed computing may be necessary to address these challenges effectively (Jain, R., & Zhai,
Q. (2021). The capabilities of AI tools must align with the unique requirements of advanced nanometer nodes. Design exploration,
yield enhancement, and power optimization demand sophisticated AI algorithms capable of handling complex IC architectures. AI models
must adapt to the rapidly evolving IC design landscape. A continuous learning approach ensures that AI algorithms stay up-to-date
with the latest process technologies and design methodologies (Sun, X., Li, H., & Xu, Y. 2021).
One important point to consider is that
despite significant progress, AI still lags behind human experts in some areas that require intuition to solve (Liu & Chakrabarty,
2021). Nonetheless, AI is improving rapidly and could become a dependable tool in the near future.
Despite the challenges, the potential
of AI technology in IC design remains promising. As AI algorithms evolve, they are expected to overcome the limitations and redefine
the landscape of IC design for advanced nanometer nodes.
Chapter Summary
The process of organizing the literature
review was taken in stages, beginning with an overview of the main topics of AI and IC design. A deeper analysis of each of the
sections was then followed with a highlight of their relation to the problem of the study. A final section on the gaps in the literature
and the concluding observations were made to bring together the literature.
AI is swiftly altering the human approach
to problem-solving in many different frontiers (J. Lee et al., 2021). Computing can take advantage of AI at the core level in the
design of chips. The identified issue that currently needs improvement is the verification stage (Fujita, 2019). This stage is
currently mostly manually performed by engineers introducing a bottleneck (Miranda, 2020). Early ML and AI models are being developed
to help counter this problem. The need for easier and more efficient design processes for custom chips is also rising. These chips
are often complex and are susceptible to attacks through hardware-based Trojans (Vashistha et al., 2018). The next stage in computer
improvement is identified as speeding up the design process for more diverse machines. The identified gaps are in easing the design
process without compromising quality, introducing low-cost solutions, and combining different technological approaches for better
solutions in chip design. This paper further explores the AI and IC design subjects through a methodological approach outlined
in the coming chapter. The following chapter after the literature review is the methodology section which outlines the steps the
dissertation is taking to explore the research questions.
Chapter 3: Methodology,
Design and Methods
This chapter describes the approach used
to complete the study by gathering the necessary information and analyzing it. The purpose of the qualitative exploratory research
was to explore the disconnection between AI applications and IC design. The problem lay with the high costs of designing chips,
difficulties in the verification of IC circuits due to the complexity of the process, and limitations in the automation of the
verification process, which modern technology had yet to achieve but intended to be handled by AI (Kovacs et al., 2020). The conceptual
framework explored the major topics of AI and ICs, dissecting them and combining different subtopics to reach a conclusion investigating
the raised issues. This detailed approach was covered by the qualitative study outlined below, which states the study’s paradigms,
philosophy, steps, and considerations.
Research Methodology and Design
The research utilized the qualitative
approach, which involved looking into non-numerical information to obtain insights from it. The qualitative research targeted handling
the IC design problem through existing or upcoming methods in AI. The choice of the qualitative approach leveraged the nature of
the topic and the intent of the study. The topics involved a few theoretical philosophies on approaching circuit design with additional
contributions of possible improvements. Using the qualitative approach was necessary to match up the phenomena behind problems
associated with integrated circuit design. The Likert scale questions helped provide a quantitative viewpoint of the participant’s
views of the topic areas. An additional reason for the qualitative study was to investigate the existing problems and attempt to
provide insights or answers regarding more efficient IC design. Qualitative research approaches also aim to simplify the complexity
of a subject and provide newer viewpoints on the issues (Martinez & Valverde, 2022). The relation of this research to the Doctor
in Computer Science program was that computing is constantly evolving as newer approaches are realized. Frequent review of the
existing solutions and the possibilities of the new ones allows upcoming solutions to be created. AI and ML are the current new
frontiers that are slowly maturing and helping to improve new IC design approaches (Mirabbasi et al., 2023).
The research design chosen, the exploratory
design, was driven by the paradigmatic interpretive perspective. This interpretive perspective and exploratory design are used
to dig into a topic and provide the necessary information to enlighten and derive meaning from it as thoroughly as possible (Doyle
et al., 2020). Looking at the need to reveal the issues surrounding IC design and AI, it was fitting to utilize the exploratory
design to explain the two topics. The results came as a summary that reveals the shortcomings and areas needing improvement. The
sources for the data included a questionnaire targeting the area of focus.
As noted, the research design selected
for this study was the exploratory design. This approach aims to look deeper into the progress so far on a topic of interest. For
this research, the topics are AI and ICs design, and which analysis was needed to move to the next revolution of computing. The
exploratory approach provided the framework to guide the data gathering and help in extracting the knowledge, expanding it, and
bumping up the progress in the topics.
This qualitative exploratory approach
was justifiable because it combined the existing approaches and views and consolidated them while adding new views and possible
solution approaches (Swedberg, 2020). This approach ensures that the contribution of the two areas is represented and the main
themes revealed. These themes allowed the formulation of the additional themes that continue the story and allow the continuation
of the story.
Population, Sample, and Participant
Recruitment
The population of the study was the experts
connected to the design of computer chips through modern approaches. This population covered the practitioners in the computing
fields who partook in creating better-performing integrated circuits. The choice for the population was to understand their impacts
and knowledge progress in improving computing through approaches like machine learning and artificial intelligence. Due to the
technical nature of the topics being studied, the population for the research had to be limited to individuals with significant
experience and important positions. Therefore, a small group was selected for the study. The size of the target population is hard
to estimate given the highly technical nature of the area. This study assumes about 50,000 population, with more than 25 years
of experience in the USA (Semiconductor Industry Association).
The target sample of the study was a set
of eight experts familiar with artificial intelligence and integrated circuit design. The reason for narrowing down the type of
participants was that the areas of study were narrow and quite technical, and a randomized group of participants would not possess
the necessary knowledge for a meaningful outcome. This group of participants was intended to provide their expert view and knowledge
on the research questions and contribute to its objectives.
The choice for participants was individuals
who have dealt directly or indirectly with the designing of integrated circuits practitioners in the artificial intelligence and
machine learning fields and possible researchers who were concerned with the progress of AI and IC design with at least five years
of experience. These characteristics made up the necessary target population and sample group to provide adequate knowledge and
allow for an expansion by studying their input.
The intended sampling approach was to
use the purposive sampling method. This is a non-probabilistic sampling approach that utilizes participants who best fit the study
(Indrayadi, 2020). The reason for choosing this approach is that the area of study was quite narrow and requires a high set of
specialized skills. There was a need to identify the possible candidate and request them for participation in the study, then administer
the information-gathering process.
Accessing the sample was not easy, given
that the target area is a niche area. Further, the target participants often find it hard to find time owing to their busy lives.
The confidence for the access of the sample, therefore, was medium with the intention to use boosting approaches through snowball.
Following Institutional Review Board approval, the starting point for recruiting the participants was my personal network of experts
in the field. These were contacted via phone calls to inform them of the intention of the study and enquire about their participation.
The goal was to have a sample size of eight participants for the survey. The phone calls informing the participants were also followed
up by an email with the invite link to the consent form. Filling out the form and confirming the details then prompted the sending
of the invite link to fill up the survey for the participants to fill out as needed.
Data Collection Instrumentation and
Procedures
The collection approach and instrument
in the study was a questionnaire. The choice of this collection instrument was to gather the sentiments and viewpoints of the participants
in relation to the topic. The usage of the questionnaire in a qualitative study was motivated by the time restrictions available.
A qualitative study can use a questionnairent
in the study was a questionnaire. The choice of this collection instrument was to gather the sentiments and viewpoints of the participants
in relation to the topic. The usage of the questionnaire in a qualitative study was motivated by the time rich
and detailed data. These methods allow researchers to explore complex phenomena, gain a deeper understanding of participants’
perspectives, experiences, and behaviors, and generate detailed narratives. However, questionnaires can be used in qualitative
research as a supplementary data collection tool in certain situations (Creswell, J. W. (2013, Denzin, N. K., & Lincoln, Y.
S. (Eds.). (2011). For this study, I chose a questionnaire that
enabled the collection of data through both open-ended and closed-ended survey questions. This approach efficiently gathered the
participants’ viewpoints and knowledge. This instrument is commonly used in qualitative studies within the field of computer
science (Kamal et al., 2020; Powe II, 2019). Consent of the participants was first required before commencing each session to gather
their views. The text filled in the questionnaires was key to interpreting and comparing the results of the participants. This
study aimed to receive the most honest opinions on AI and IC design in the modern world. Integrated circuits (ICs) are at the heart
of many modern electronics, powering everything from smartphones and computers to medical devices and automobiles (James et al.,
2022). IC design is a complex and time-consuming process, requiring expertise in circuit design, simulation, and layout. As of
2023, AI has been employed to design ICs, promising to improve the efficiency and speed of the design process. AI technology is
often used to design modern integrated circuits design due to its substantial benefits. The participants, therefore, were encouraged
to be as detailed as possible and try to keep their responses unbiased. The choice of the questionnaire was to allow as much time
as possible for the participants to internalize the questions and answers based on their experiences and knowledge. This means
that they could also pause and resume the answering at a future date as long as it was before the deadline for providing the answers.
Raw data points were also available for the replicability of the study.
After signing the consent form, the participants
were then provided with a link to connect to the Google Forms platforms for the questions (see Appendix A). This link is redirected
to Google Forms with the survey questions, which the participants had to go through and answer. Each of the unique IDs of the participants
was used for identification purposes. The data was temporarily held in the Google account containing the form pending the filling
of the participants. This data was then exported for further analysis through Excel.
Data Analysis Procedures
The initial step covered the gathering
of the survey responses into a dataset for easy analysis. While Google Forms provided minimal tools to create visualizations, the
dataset needed exporting for deeper analysis. Data exploration and cleanup were then conducted on the data to understand its structure
and rectify any mistakes available (Foxwell & Foxwell, 2020). The exploration also involved the creation of additional visualizations
to better understand the responses.
The text mining and analysis process covered
the translation of the information obtained in the research so far into meaningful code for easy interpretation (Richards &
Hemphill, 2018). The process took place in two stages, where an initial set of interpretations and codes were applied and then
refined to the final code in the last stage. The coding process was manually handled with support and help from Microsoft Excel.
The results were interpreted to get the general knowledge contributed by the participants and its relation to computing in general.
Qualitative analysis to determine the main themes, patterns, insights, and knowledge from the data was conducted. This allowed
the triangulation of the results to pinpoint the validity of the knowledge about the research. Finally, these insights were merged
into a collection of insights and summary points on the research for the final reporting process.
Trustworthiness
Trustworthiness in the research provides
affirmation of why the proceeds and the results should be regarded as important to expand the knowledge. Trustworthiness generally
covers four main areas, which include credibility, transferability, dependability, and confirmability (Kyngäs et al., 2020).
Credibility in this study is assured by
sourcing the information from areas that are known to produce truthful information (Self & Roberts, 2019). Going through the
general contribution of the involved information allowed the differentiation of credible sources, with the ones not trusted eliminated.
The participants providing the responses were required to be highly skilled in the area where they provided the information. The
questionnaire and the picking of the participants concentrated on the experience level of each participant to back up their contribution.
The study also involved triangulation to support its credibility. The process covered the combination of sources and approaches
to improve the level of credibility of the study (Noble & Heale, 2019). The process further involved the comparisons of the
gathered responses in a bid to improve the understanding of the researcher to make credible additions to AI and IC design. To use
triangulations effectively, the researchers gathered data from the survey of multiple participants and combined it with the existing
literature gathered so far on the topics.
The information and results collected
from the questionnaires have to be easily transferable to the study to reveal insights (Kyngäs et al., 2020). Ensuring this
characteristic was enforced by keeping the questions straight and to the point and the topics specific. This eased the complexity
of the information and reduced the chances of ambiguity during the process of transferring the data. The population targeted in
the study were the industry experts handling the design of IC chips. Additional targeted experts were also those who utilized artificial
intelligence and machine learning to solve problems. This means that the contribution was expected to expand their knowledge while
gathering what they understand so far. The research was also set to utilize the latest technology in gathering and analyzing the
data to convert it to knowledge and for use in making conclusions.
Dependable data is necessary for expanding
the topic areas. Ensuring dependability is addressed by linking the study with relevant data on the topic areas (Sumrin & Gupta,
2021). All steps are also outlined in the process conducted without hidden critical choices. Openness ensures that the study can
be investigated and reproduced as needed. The research also ensured that the data was constant and not altered in any way by limiting
the people interacting with it. The gathering tools were also limited, with only access to the researcher to view and interact
with the responses. The procedure of transferring was also keen to keep the data in its original state by limiting the path of
transfer between different tools.
To allow the study to be confirmable,
the measures taken need the adequate linking of relevant and accurate sources (Stenfors et al., 2020). Tracing the information
and verifying it ensured that it is as reliable as possible. The researcher intended to be neutral at all times when interacting
with the responses. Any contribution from the participants was interpreted as is and included in the conclusions without change.
The trail of contribution was also recorded for any auditing necessary after the data gathering was done. Bias was eliminated,
and any piece of data was removed from the analysis and interpretations.
Ethical Assurances
Protecting the participants was performed
by handling the collection process as private. The research did not collect personal information and placed the participants in
a position where they would not provide their views freely. Any sensitive information that might be captured was redacted and removed
from the collected dataset. The participants also had the freedom to back out of the data-gathering at any point in the collection
process. The participants had to consent to the study before starting the entire process.
This consent was relevant to the Belmont
report in that it provided informed consent, outlined the risks and benefits, and had a fair selection of the participating subjects
(Sims, 2010). The study was set to maintain the high respect of the people contributing to its knowledge base. Their contribution
was acknowledged and highly respected by thanking them and following up on the impact of their contributions. The research also
intended to be and uphold laws and rights for the participants and those affected by the results. The researcher had a moral obligation
to ensure the best outcome for the participants and the research. This call for beneficence made sure that the core part of the
research and the researcher was to do good by expanding knowledge and contributing to problem-solving. The research also benefited
society in that it sought better problem-solving through improved computers.
Data will be stored for a maximum of seven
years through encrypted archives only accessible to the researcher. For permanence, the data was replicated after encrypting to
copies of the archives and future retrieval if possible. The research was also reviewed by the CTU Institutional Review Board to
ensure that it keeps up with its claims and adheres to high ethical standards.
Chapter Summary
This chapter has indicated the method
applied to the dissertation. The study focused on using a qualitative approach through an interpretivist paradigm. This framework
allowed the study to provide a narrative of the topic areas, seeking the gaps and outlining possible solutions. The study described
the topic in detail and revealed the available issues. The instruments used were a survey through a questionnaire. Raw data was
provided for the replication process, with results presented through summaries.
The trustworthiness of the study was ensured
by using reliable sources, especially skilled participants in the relevant professions. The study also ensured dependability by
being straight to the point and providing enough details for easy interpretation and understanding. All participants were taken
through a process to consent and given room to back out on uncomfortable areas.
Chapter 4: Findings
This chapter handles the presentation
of the results of the study. To recap, the purpose of this qualitative exploratory research was to explore the disconnection between
AI applications and IC design. The major problem lies with the high costs of designing chips, difficulties in the verification
of IC circuits due to the complexity of the process, and limitations in the automation of the verification process, which modern
technology is yet to achieve but intended to be handled by AI (Kovacs et al., 2020).
Description of the Study Sample
The population of the study was the experts
connected to the design of computer chips through modern approaches. They ranged in age from 48 to 65 years old. Four participants
were from the USA. One participant was from Canada. Three participants were from Israel. Years of experience in computing ranged
from 28 to 39 years.
This population covered the practitioners
in the computing fields who partook in creating better-performing integrated circuits. This population was relatively small, given
the technical nature of the topics. The estimated size of the target population was roughly 50,000 worldwide, given its complexity
and niche status.
The target sample of the study was a set
of eight experts familiar with artificial intelligence and integrated circuit design. The reason for narrowing down the type of
participants was that the areas of study were narrow and quite technical, and a randomized group of participants would not possess
the necessary knowledge for a meaningful outcome. This group of participants was intended to provide their expert view and knowledge
on the research questions and contribute to its objectives.
The choice for participants were individuals
who have dealt directly or indirectly with the designing of integrated circuits practitioners in the artificial intelligence and
machine learning fields and possible researchers who are concerned with the progress of AI and IC design with at least 5 years
of experience. These characteristics make up the necessary target population and sample group to provide adequate knowledge and
allow for an expansion by studying their input.
The sampling approach used was the purposive
sampling method. This is a non-probabilistic sampling approach that utilizes participants who best fit the study (Indrayadi, 2020).
The reason for choosing this approach is that the area of study is quite narrow and requires a high set of specialized skills.
There is a need to identify the possible candidate and request them for participation in the study, then administer the information-gathering
process.
The final sample size used for the study
was eight participants, with the choice coming from the similarity of the responses to provide a clear and meaningful contribution.
These were all experts with decades of experience in artificial intelligence and integrated circuit design. All eight participants
were male and above 45 years of age. This means that they were people with experience in computing. The years of experience were
all above 25 years, with the most being 39 years in the industry. The participants were from USA, Israel, and Canada. It is also
notable to point out that all the participants had knowledge of computing and integrated circuits. In the latter area of focus,
the participants also had experience and knowledge of the verification process in circuit design.
Results
The questionnaire posed 20 questions to
elicit the expertise of the participants to address the central research question for the study, how can AI be implemented within
IC design, and if so, what are the options? The questions asked are listed below:
| 1. | How do you rate yourself on understanding AI? |
| 2. | How do you rate yourself on understanding IC design? |
| 3. | What level of disruption is likely to occur to IC design from AI? |
| 4. | How will this disruption occur if you think it will happen? |
| 5. | How relevant is Moore’s law today? |
| 6. | Is Moore’s law dead in your opinion? Why So? |
| 7. | What level is AI likely to influence IC design in the future? |
| 8. | What is the likelihood of AI-based IC design overtaking engineer-based IC design? |
| 9. | How will IC design change over time in the coming future? |
| 10. | Do we currently need customized chips to fully support AI and ML? Why so? (For your choice above) |
| 11. | How useful is the contribution of AI in developing better computer designs across: |
| 2. | More powerful computers? |
| 12. | How likely is AI to provide solutions for IC design? |
| 13. | Do you have knowledge of the verification process of IC design? |
| 14. | How likely will AI help improve the verification process of IC design? |
| 15. | How likely are AI approaches to take over IC design verification? |
| 16. | In a few words, what is your opinion on AI improving IC design? |
| 17. | What are the key areas necessary to integrate AI automation in IC design? |
| 18. | How likely is it to keep advanced IC design costs low? How so? (Feedback) |
| 19. | Do we generally need so many powerful computers? Why? |
| 20. | Do you have any other relevant information/insights about AI Technology involvement within future IC design? |
The insight per question is presented
to follow. Each question result is presented in sequential order to demonstrate the different levels of complexity applied in the
study. The questions progressed from general ones to more detailed ones and thus preferred to be presented in their sequential
order. The information from the questions not presented in the results was integrated into the results and the interpretations,
as their nature makes it hard to report them out rightly. This knowledge was integrated to ensure the continuous and smooth reading
of the paper.
Technological Disruption
Question 4 asked, how do you anticipate
the world’s technological disruption to occur if you think it will happen? The general consensus regarding the oncoming disruption
through technology is that computers will get more advanced, especially in artificial intelligence. This perspective is demonstrated
by the response, “As new ICs are much more complex to design, it becomes more challenging to design and manufacture modern
ICs. The field will need more automation software advancements, which will be definitely AI based.” The participants
pointed out that AI is rising rapidly alongside the complexity of integrated chips. Chips are also becoming smaller, as reported
by one of the participants, with their integration spreading to almost all areas of life. Designs are also set to get more complex
and result in more complex computers that might be beyond our current understanding.
The Relevance of Moore’s Law
Question 5 asked, how relevant is Moore’s
law today? And question 6 asked, in your opinion, is Moore’s law still a standard in the IC arena? Explain? Five of the eight
participants reported that Moore’s law is still relevant at the present time at a ranking of high likelihood. The remaining
three reported a neutral view of Moore’s law being relevant currently. The views from the participants indicated that Moore’s
law still exists but not in the sense it was first defined. As an example, one participant responded, “Not sure. Technologists
no longer shrink the transistors the same way they did in the first decades since Moore introduced his law. The geometry scaling
has now different meaning.” The participants are of the view that transistors are no longer shrunk in the manner
that they were in early computing days. Other participants observed that the transistors are more than doubling every 2 years,
while others think that the law has finally reached its peak.
The Future of IC Design
Question 7 asked, what level is AI likely
to influence IC design in the future? Seven of the eight participants agree that the likelihood of the future of IC design being
influenced by AI is high. One participant had a neutral standpoint on the matter.
AI-based Design
Question 8 asked, what is the likelihood
of AI-based IC design overtaking engineer-based IC design? The participants had a divided view on the likelihood of AI overtaking
engineers in designing integrated circuits. 5 of the eight rates the takeover as likely and very likely. One participant had a
neutral standpoint on the takeover, while the remaining two see that the takeover is unlikely.
IC Design Change
Question 9 asked, how will IC design change
over time in the upcoming future? Integrated circuit design changes over time seem to be getting more complicated, as indicated
by six of the eight participants. According to the participants, these chips are set to include more features in computation. One
noted, “IC design and manufacturing will have to include more advanced technologies in order to make more complex chips.”
The participants also pointed out that the chips are set to use less power despite their increase in complexity. Their integration
with AI also seems to be a direction some participants pointed towards. Automation will also be more widespread as these chips
become tinier and more complicated. However, two participants pointed out that the changes will not be as drastic as anticipated.
The already tiny 3nm circuits are almost at their physical limit, and there might be no more advances.
Customized Chips
Question 10 asked, do we currently need
customized chips to fully support AI and ML technologies? Seven of the eight participants agree that we need customized
chips to support AI and machine learning technology fully, highlighting things like, “It is probably proven performance
gain over use non- specialized chips. I mean, there could be the data to support it.”, and “To achieve high
computing power, keeping design time lines in a reasonable range, maintain accuracy and compliance with complex deep nanometer
design rule complexities. “. They pointed out that more complexity and higher computing power cannot be solved by existing
chip designs. The participants also noted that custom chips are more optimized for handling specific tasks yielding better results.
Adjustments in the architecture of the chips is a notable change that the participants agree needed to happen to accommodate the
needs. However, one participant disagreed and pointed out that it is not necessary for the changes.
AI and Better Microchips
Question 11 inquired about the level of
contribution of using AI in the development of improved microchips, which was measured through nine options in Figure 2. Different
technologies are seen to be of varying importance in introducing AI to IC design. It appears that most participant agree that AI
will have a significant impact on Synthesis, SoC, ASICs, and Memory ICs. This is because these fields rely heavily on IC Design
automation. However, there is less predicted involvement of AI technology in Analog, MEMS, Photonics, and other areas. This is
likely because these domains require more human expertise that is based on extensive experience and knowledge. The varying levels,
as presented, show that there is a high likelihood of newer ways to create microchips in the coming future.
Figure 2
Question 11 Results.
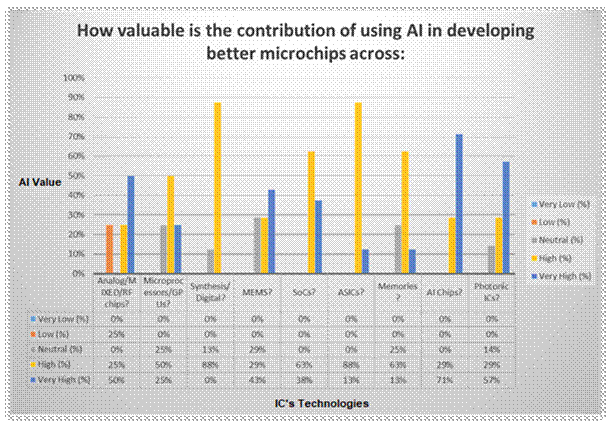
AI Solutions for IC Design
Figure 3
Question 12, 14, and 15
results
In Figure 3, there is analytics provided
for questions 12, 14, and 15. These questions were designed to collect information on how AI technologies will impact the IC design
field in the future. The results show that participants are in agreement that AI will have a positive impact, allowing for advanced
solutions and significant design improvements.
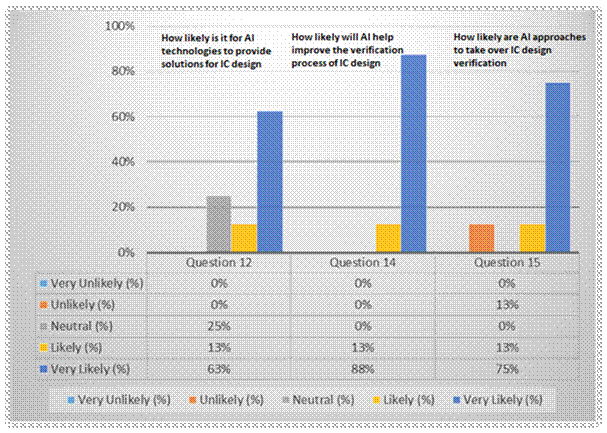
Question 12 asked, how likely is it for
AI technologies to provide solutions for IC design? Concerning the likelihood of AI providing solutions to IC designs, six
of the participants agreed that it is likely for AI technologies to provide solutions for integrated circuit design. The remaining
two had neutral standpoints on such contributions.
On investigating the likelihood of AI
improving the verification process (Question 14), the participants also universally agreed that artificial intelligence contributes
to improving the verification process in integrated circuit design.
Question 15 asked, how likely are AI approaches
to take over IC design verification?, seven of the eight participants agreed that it is very likely that AI-based approaches will
take over the verification of integrated circuits. The participants pointed out that the complexity might be too high for an engineer
to carry out, as pointed out by one participant said: “AI is the only way to verify a deep nanometer IC of 5nm and below.
It will be used for functional verification, physical verification, simulation and manufacturing aspects like DFM analysis”.
These changes are expected to happen in the near future as AI will easily go through the existing approaches and find the best-fitting
ones for different tasks. One participant highlighted that it is unlikely for AI to take over. He pointed out regarding AI that,
“It can suggest improvements that will need to be verified by an Engineer”.
Question 17 asked, what are the key areas
necessary to integrate AI automation in IC design? and the participants quoted that it varies from the architecture, the verification
processes, validation, physical design, and manufacturing. One said, “Physical verification, Simulation, functional verification,
Design For Manufacturing (DFM) analysis, Reliability Verification (RV), DRC, LVS”, and another pointed out the areas
as “Manufacturing, high level architecture, functional verification, physical verification, DFM considerations.”
Question 18 asked, how likely is it to
keep advanced IC design costs low using AI Technology? The participants observed that the design costs for integrated circuits
have a high likelihood of being kept low through AI, as six of the participants indicated. These opinions came from the understanding
that AI technology will improve automation and require fewer human resources and costs. The remaining two were neutral regarding
the improvement of the costs through AI.
Additional Opinions on AI
Question 19 asked, how do you see the
involvement of AI techniques within Quantum Computing ICs? The participants observed that the complexity contributed by AI is expected
to be huge in regard to quantum computing. One participant said, “Definitely. Due to the complexity of Quantum Computing
process, AI is most likely the only way to perform it. AI is the only technology that will enable vast, intelligent computing power
and resources distribution efficiency which is a key in Quantum Computing”. Five of the eight participants did not have
knowledge or had neutral thoughts on AI having a significant contribution to quantum computing.
Question 20 asked, do you have any other
relevant information/insights about AI Technology involvement within future IC design? The sentiments were that it is set to be
common for the computer creation process. Some participants are of the view that this technology is set to dominate computing in
the near future, saying “AI will become a common technology for IC design firms. EDA tools will incorporate them in many
ways to increase IC design’s performance, productivity and accuracy.”, and “AI will eventually
be able to automatically design microchips from beginning to end. We will reach the situation of computers-making-computers.”
Overarching Findings
While participants agreed that AI is
set to change computer design, there was a divided opinion on whether AI will likely be a revolutionary change in chip design or
not. Despite the lack of consensus on whether AI will be revolutionary to changes in chip design, the aggregated results from the
study indicate several key findings.
IC design keeps getting complex with the
increasing demand for computer tasks. The complexity is prompting the inclusion of AI to help in designing the chips. Upcoming
concepts such as deep learning are set to improve the design process and will soon take over the design processes.
AI will also bring about far-reaching
changes, such as improving automation, excelling in repetitive tasks, solving problems requiring high computing, and pointing out
possible solutions.
There are physical limitations to chip
design and improvements are being worked on through artificial intelligence. Further, the design processes might be currently at
their maximum operations needing a technological breakthrough.
Discussion of Study Findings
Through the analysis of the data collected
from the study participants, the key findings emerged to include AI is unavoidable, automation is needed to improve the performance
of IC design, Moore’s law might be on its end times, and AI is set to be valuable in many of the processes in IC design.
Through these findings, the central research question was addressed. The central research question for this study asked, how can
AI be implemented within IC design, and if so, what are the options? The theme of AI being valuable to many of the processes in
IC design provided insight into the options for the question. AI is set to help in suggesting solutions, helping in the development
of chips, processors, verifications, IC integration, and more. The theme of AI being unavoidable illustrates that technology is
a major change that infiltrates all areas. It is clear that AI will help in changing our approach to IC design and will provide
useful insights and alternative viewpoints.
The general agreement by the participants
that AI is set to change the realm of computer design shows that it is here to stay. This is supported by Li et al. (2019) observation
of AI being a major changing point in technology. However, there was also the theme of some skeptical sentiments among some participants
who view that technology might be overhyped. Therefore, there was a divided opinion on whether AI will likely be a revolutionary
change in chip design or not.
Chapter Summary
The contribution of the participants was
a good one as far as AI and IC design are concerned. The participants had good knowledge and experience in the area, which was
required by the study. This knowledge was also highlighted in the quality of the answers, which were good. The purpose of the study
was to look at the options available in AI and IC design was well represented by the participants upon observing the results. The
implications of the study findings are evaluated in the following chapter.
Chapter 5: Discussion
and Conclusions
This chapter highlights the discussion
of the presented results and provides a conclusion to the study. The purpose of this qualitative exploratory research is to explore
the disconnection between AI applications and IC design. The problem lies with the high costs of designing chips, difficulties
in the verification of IC circuits due to the complexity of the process, and limitations in the automation of the verification
process, which modern technology is yet to achieve but intended to be handled by AI (Kovacs et al., 2020).
Limitations of Study Findings
One of the key limitations is that the
chosen participants were all experienced and had decades-long interactions with computing. While this was an added advantage to
the study, it means that the likelihood of bias in their views was high. Continued working in the industry means that they may
be more likely to be resistant to any changes. This can be supported by the hesitance to acknowledge AI as a replacement and consider
it a helper technology. Having such a limitation in mind is necessary for interpreting the sentiments provided by the participants.
A wider range of participants would have been better, but challenging to identify and reach out given the highly technical nature
of the area of study.
Another limitation of the study is towards
challenges in the sampling process. It was hard to find new experts in the field to partake in answering the questions. This left
the study with highly experienced participants only. The balance needed for a study was not accomplished in this case. The gap
was filled by going through other studies and including their knowledge in this paper and incorporating the interpretations of
the participants with the gathered information. The study is still useful despite this limitation, as the literature review covers
the gap by including updates on information that complements the contribution of the participants.
The sample size of eight participants
might also have been small for the study. A wider number of participants would cover more areas and provide a more detailed view.
However, a small sample size is appropriate for qualitative exploratory studies (Shaheen & Pradhan, 2019). Data saturation
was observed in the participant responses. This small sample size is also a key part of knowledge to have when going through the
paper and trying to understand the interpretations. However, the study is still beneficial in that few participants had valuable
knowledge with decades of experience. This means that their contribution was deemed necessary in the changes upcoming in AI and
IC design.
Interpretation of Study Findings
The responses from the participants make
it clear that artificial intelligence is changing integrated circuit design. This means that it is a technology that will stay
around for a long time and influence the approach to creating computers in the future. This view is confirmed as highlighted by
Berente et al. (2021) illustrated in the literature. One of the ways it is set to help in IC design is by suggesting solutions
during the design process.
Despite the lack of consensus on whether
AI will be revolutionary to changes in chip design, the aggregated results from the study indicate several key findings, including
the unavoidability of AI, the improvements in IC design, and Moore’s law being towards its end. Another finding is that AI
will bring about far-reaching changes, such as improving automation, excelling in repetitive tasks, solving problems requiring
high computing, and pointing out possible solutions. AI will improve solutions by looking for vast datasets and gathering intelligence
while comparing multiple designs for the best ones. For customization, the multiple designs will be useful in tuning specific areas
of the circuits to fit the needs. Monroe (2018) confirms this integration of AI into IC designs for custom situations. AI is also
set to help in the verification of designs which is considerably a bottleneck in the current processes. Computerizing this process
is expected to introduce a whole new way of viewing the design of integrated circuits. Automation is also a strong candidate for
the contribution of AI in IC design. Most processes are repetitive and would fit well into automation. Adding AI means that the
automation will be intelligent and improve over time as issues are discovered and solved. Current automation is limited to robotics,
and introducing AI is among the top needs for manufacturing processes. AI is also good at offering alternative viewpoints. Its
interaction with knowledge bases means that it can gather information and formulate alternative ways to handle issues. The suggestions
will be good in challenging designers and lead to better circuits.
A good consideration is that artificial
intelligence is a helper to design experts. Its development is not advanced enough to completely replace them. The design experts
also have human intuition in handling the designs, a feature that AI might be lacking. This is in contrast with the observation
by Nardi et al. (2019) that AI could replace most positions through automation. Incorporating processes that utilize the expertise
of the designers and the capabilities of AI will therefore lead to better circuits. This is promising for fields where custom chips
are necessary and where human expertise and AI will play well. The changes are also set to introduce more possibilities for research
in the area and further extend the capabilities of computing.
Regarding Moore’s law, it is observed
that it is no longer applicable as much as modern changes are concerned. Transistors in chips are now too dense to continue doubling
every two years. The current level of density of transistors has also surpassed the expectations of the law. This invalidates it
with regard to its original view and interpretation. The widespread availability of powerful small devices is a testament to Moore’s
law, where circuits have gotten small and heavy computation is possible in limited spaces. A considerable view is that such power
might be at its peak, given the limitations in the capabilities of the density of the transistors.
Practice Implications of Study Findings
This study reveals that AI is capable
of helping out in integrated circuit design. This help comes in the form of working out efficiencies in repetitive tasks or suggesting
improvements in the design process. These contributions imply that AI should be integrated into IC design as soon as possible to
start helping out in the process. Integration could involve experiments to try out the suggested improvements while monitoring
the performances. AI is also an area that requires a lot of testing in several phases, and starting out translates to results being
implemented in the near future. Documenting the results of the experiments can also help in iterations on the improvement of the
design processes aided by AI.
So far, the progress in computing and
chip design has introduced a wide range of possibilities for creating integrated circuits. Each of these design approaches has
its own upsides and downsides. Having a way to collect the design processes and feed them into an AI system can be a start in commencing
the improvements. Since AI works best when trained with large datasets and allowed to seek better approaches, using these design
processes can be a good start. The system can also do well with input on how these designs have changed and what is expected, and
how the design processes have contributed.
The study’s revelations on the capabilities
of AI point to the needed bridging of computing with other fields. With the needed custom chips for different activities, it would
require input from both ends to design the perfect chips. This is also an area where AI is set to contribute to by analyzing these
custom needs and incorporating the knowledge in the designs.
One action is to open up the research
on AI and other fields to as many individuals as possible. One recurring theme in technological revolutions is the existence of
intensive research prior to the implementation of the technologies. Such research ensures that all theoretical possibilities are
explored before the experimentation starts on the capabilities of the technology. For IC design, expanding the research to the
non-technical fields is set to help reveal the possibilities, problems, and how each of the areas complements each other. Better
solutions are, therefore, expected when the research area is as accessible as possible to people contributing.
Researcher Reflections
Looking at the study reveals key takeaways
that have shaped the answers it intended to find. For instance, the intricate nature of IC design is a key part of driving computing.
Circuits are complex objects that need attention to detail and require many processes. The view towards integrated circuits has
changed over the course of this research study and highlighted their critical nature. The existence of circuits in many electronic
devices goes to show how these designs affect everyone. It is also important to highlight that IC design needs experts during the
creation process. This clears up the doubt present on whether AI is to replace the designers. The complexity of the circuits is
too much to be currently handled by AI, which points to the fact that their input will remain invaluable for a long time to come.
Integrated circuits, therefore, make up the core of computing, and designing them is the start of their usefulness.
Regarding artificial intelligence, there
is a clear view that it still has a long way to go. The initial view was that it was nearly taking over computing tasks. This view
has so far been updated to that AI still has some evolution to get to a state where it can be dominant. The state of AI is that
of a helping technology, and it is evolving to be more complex. This also brings the view to AI being unstoppable in most tasks.
Tasks requiring repetition, knowledge from large sets, and alternative viewpoints will benefit greatly from AI. The insight from
the participant has helped reshape the notion that AI will remain as a limited helper to humanity. Its contribution to computing
alone is enough to change the view towards our interaction with computers. AI-powered chips and machines, therefore, are about
to be implemented more.
Recommendations for Further Research
There is a need to research more into
artificial intelligence and integrated circuit design. This research reveals that there is more to these two areas which can be
explored and which should be handled through an exploratory qualitative study. The research can look into both the theoretical
and practical aspects of the areas.
Another recommendation is to look into
consolidating the integrated circuits design knowledge. The limited information currently on the area shows that it can take a
lot of work to seek its knowledge. Its consolidation can help fuel further research onto how they work and how they can be improved.
This is possible through an extensive literature review on the fields.
A quantitative study into the extent of
the IC designs and how well they can be improved by AI is also needed. Research can help in revealing how much work has been accomplished
so far and what is needed for the next leap in revolution, paving the way for more.
The last recommendation is towards the
nature of IC designs. There is a need to look at the options available in automating IC design. This phenomenon was beyond the
scope of this study and is needed in future research. It can help reveal the possibilities and what steps need to be taken through
a qualitative study.
Conclusion
The purpose of the qualitative exploratory
research is to explore the disconnection between AI applications and IC design. The problem lies with the high costs of designing
chips, difficulties in the verification of IC circuits due to the complexity of the process, and limitations in the automation
of the verification process, which modern technology is yet to achieve but intended to be handled by AI (Kovacs et al., 2020).
The research question centers on looking for the ways that AI can combine with IC design to handle the problems experienced by
modern design processes. It is, ‘How can AI be implemented within IC design, and if so, what are the options?’. The
research utilizes the qualitative approach, which involves looking into non-numerical information to obtain insights from it. The
qualitative research targets handling the IC design problem through existing or upcoming methods in AI.
The observations of this study are quite
applicable to the computing world. For instance, the observed growth of AI means that designers and engineers need to keep improving
on the technology and find ways to integrate it. AI is here to stay, and early preparations will see solutions created as soon
as needed. The crucial nature of engineers and experts in IC design also means that they have work to do. It is clear that having
more experts contributing to integrating AI will be better and lead to well-performing machines. Experts have an obligation to
work in creating the needed knowledge base for creating AI-based chips. They also need to work with experts in other fields to
create custom chips in the coming future. The highlighted areas where AI is set to help in IC design include physical verification,
validation of the designs, the design process itself, and manufacturing and automation tasks. Some of these tasks also have repetitive
processes which will fit the AI integration and will see to better designs.
The recommendations show that there is
a need to research more into artificial intelligence and integrated circuit design. This research reveals that there is more to
these two areas which can be explored and which should be handled through exploration. The research can look into both the theoretical
and practical aspects of the areas. Another point is to look into consolidating the integrated circuits design knowledge. The limited
information currently in the area shows that it can take a lot of work to seek its knowledge. Its consolidation can help fuel further
research into how they work and how they can be improved. A quantitative study into the extent of the IC designs and how well they
can be improved by AI is also needed. Research can help in revealing how much work has been accomplished so far and what is needed
for the next leap in revolution, paving the way for more. There is also a need to look at the options available in automating IC
design. This phenomenon was beyond the scope of this study and is needed in future research. It can help reveal the possibilities
and what steps need to be taken.
The future of AI technology within the
IC Design field holds immense potential for transformative advancements and paradigm shifts. As AI continues to evolve, its integration
into IC design processes is expected to bring about significant improvements in efficiency, performance, and innovation. AI-driven
design automation will likely streamline complex tasks, such as logic synthesis, placement, and routing, reducing design time and
human intervention. This will pave the way for faster and more cost-effective IC development. Moreover, AI’s predictive
capabilities will play a pivotal role in optimizing IC designs for performance and power efficiency (Ghosh, A., Balakrishnan, P.,
& Reinman, G. 2020). Machine learning algorithms will enable precise modeling and fine-tuning of circuit behavior, leading
to highly reliable and energy-efficient semiconductor solutions. The IC Design world will also witness the benefits of AI
in analog and mixed-signal circuit design, where AI-driven simulations and optimizations will enhance accuracy and reduce design
iterations. Design verification, traditionally a time-consuming process, will be bolstered by AI’s coverage analysis
and bug detection capabilities, ensuring high-quality and dependable ICs (Mittal, N., & Vrudhula, S. 2019).
AI’s ability to explore vast design
spaces will foster creativity and ingenuity, unlocking novel solutions and pushing the boundaries of what is achievable in IC design. While
the future of AI in IC Design is promising, it also raises the importance of addressing challenges related to data security, ethics,
and interpretability. Ensuring the robustness and trustworthiness of AI models will be crucial to building reliable and safe ICs. As
the synergy between AI and IC Design grows stronger, collaboration among experts in AI, semiconductor technology, and electronic
design automation (EDA) will be pivotal to unleash the full potential of AI-driven innovations. The future of AI technology within
the IC Design field is poised to revolutionize the semiconductor industry, empowering engineers with powerful tools, accelerating
design cycles, and ushering in a new era of efficiency, reliability, and cutting-edge IC solutions (Cao, Y., Cong, J., & Zhao,
Y. 2018). With responsible and innovative AI implementation, the IC Design world is on the brink of a transformation that will
shape the technology landscape for years to come.
References
Afacan, E., Lourenço, N., Martins,
R., & Dündar, G. (2021). Machine learning techniques in analog/RF integrated circuit design, synthesis, layout, and test.
Integration, 77, 113–130.
Kilby, J. S. (1976). Invention of the
integrated circuit. IEEE Transactions on Electron Devices, 23(7), 648-654.
Todorov, I. and Dabral, S. (2020). Machine
Learning and AI in ASIC Design Automation: A Comprehensive Survey. In: Proceedings of the 57th ACM/IEEE Design Automation Conference,
pp. 1-6.
Hutchins, W. J., Diehl, V. A., Diersen,
M. A., & Wheeler, K. R. (2021). Large language models: Too big to fail?
Dosovitskiy, A., Beyer, L., Kolesnikov,
A., Weissenborn, D., Zhai, X., Unterthiner, T., ... & Houlsby, N. (2020). An image is worth 16x16 words: Transformers for image
recognition at scale.
Haarnoja, T., Zhou, A., Abbeel, P., &
Levine, S. (2018). Soft actor-critic: Off-policy maximum entropy deep reinforcement learning with a stochastic actor. In International
Conference on Machine Learning (pp. 1856-1865).
Finn, C., Abbeel, P., & Levine, S.
(2017). Model-agnostic meta-learning for fast adaptation of deep networks. In Proceedings of the 34th International Conference
on Machine Learning-Volume 70 (pp. 1126-1135).
Chen, T., Kornblith, S., Norouzi, M.,
& Hinton, G. (2020). A simple framework for contrastive learning of visual representations.
Kipf, T. N., & Welling, M. (2017).
Semi-supervised classification with graph convolutional networks. In International Conference on Learning Representations (ICLR).
Vaswani, A., Shazeer, N., Parmar, N.,
Uszkoreit, J., Jones, L., Gomez, A. N., ... & Polosukhin, I. (2017). Attention is all you need. In Advances in neural information
processing systems (pp. 5998-6008).
Brown, T. B., Mann, B., Ryder, N., Subbiah,
M., Kaplan, J., Dhariwal, P., ... & Amodei, D. (2020). Language models are few-shot learners. arXiv preprint arXiv:2005.14165.
Jiang, L., & Yu, Y. (2021). Analog
circuit synthesis with mixed-integer programming and machine learning. Proceedings of the 2021 Design, Automation & Test in
Europe Conference & Exhibition (DATE), 1-6.
Guo, Y., Liu, S., & Huang, T. (2020).
An advanced deep learning method for physical layout generation in digital IC design. IEEE Transactions on Computer-Aided Design
of Integrated Circuits and Systems, 39(12), 4305-4315.
Al-Jawaheri, Y. R., & Sharif, B.
S. (2019). Placement and routing of FPGAs using genetic algorithm. International Journal of Engineering Research & Technology
(IJERT), 8(11), 685-688.
Jin, W., Li, Q., Zhang, H., & Yang,
J. (2021). An automated requirement analysis method for improving software development efficiency. Information and Software Technology,
134, 106566.
Azizimazreah, A., & Esmaeilzadeh,
H. (2021). MORL: Model-based Reinforcement Learning for Efficient Automated Circuit Design Space Exploration. Proceedings of the
2021 Design, Automation & Test in Europe Conference & Exhibition (DATE), 1-6.
IEEE, Vol. 82, No. 5, May 1994 DOI: Alberto
L. Sangiovanni-Vincentelli, Rob A. Rutenbar, and L. Richard Carley Title: “The Impact of Electronic Design Automation”
Mohanty, S., Chakrabarti, S., & Roy,
K. (2014). On the performance and design of hardware classifiers using machine learning. IEEE Transactions on Computer-Aided Design
of Integrated Circuits and Systems, 33(6), 896-909.
Liu, Q., Jiang, H., Yu, X., Wu, D., &
Zhang, X. (2019). EDA: A machine learning-based efficient design automation flow for approximate computing. IEEE Transactions on
Very Large Scale Integration (VLSI) Systems, 27(7), 1626-1639.
V. Verma, N. Sherwood-Droz, P. Dong, “Inverse
design in photonics,” Nature Photonics, 14, 1–10 (2020).
John McCarthy, Stanford University –
2007 - WHAT IS ARTIFICIAL INTELLIGENCE? - whatisai.pdf (stanford.edu)
Gartner Glossary - What Is Artificial Intelligence
(AI)? | Gartner
Forbs – Innovations - The 7 Biggest
Artificial Intelligence (AI) Trends In 2022 (forbes.com)
Wikipedia – GPT 4 - GPT-4 - Wikipedia
Semiconductor Engineering -AI Adoption
Slow for Design Tools) - AI Adoption Slow For Design Tools (semiengineering.com)
Kapoor, A., Lin, R., & Green, W. M.
(2019). Power, complexity, and scaling of photonic integrated circuits. Applied Physics Reviews, 6(3), 031307.
Wong, K. S., & Mitra, S. (2019). Designing
Efficient Neural Networks through a Human-Centric AI. In Proceedings of the 56th Annual Design Automation Conference (pp. 1-6).
Tajik, A., & Samavati, H. (2019). Toward
automated analog integrated circuit design using generative adversarial networks. IEEE Transactions on Computer-Aided Design of
Integrated Circuits and Systems, 38(12), 2521-2533.
www.cadence.com
www.synopsys.com
www.siemense.com
Ghosh, A., Balakrishnan, P., & Reinman,
G. (2020). Reinforcement Learning-Based Design Space Exploration for Digital Circuit Optimization. In 2020 57th ACM/IEEE Design
Automation Conference (DAC) (pp. 1-6).
Cao, Y., Cong, J., & Zhao, Y. (2018).
Deep learning for chip design: From physical optimization to behavioral modeling. Proceedings of the IEEE, 106(9), 1616-1631.
Mittal, N., & Vrudhula, S. (2019).
Machine learning and artificial intelligence in VLSI. Proceedings of the IEEE, 107(1), 156-176
Cao, Y., Chen, T., Zhang, X., & Zhang,
J. (2020). Automated Analog Circuit Design: A Survey of Challenges, Advances, and Opportunities. IEEE Journal on Emerging and Selected
Topics in Circuits and Systems, 10(2), 215-230.
Talati, N., & Saha, I. (2020). The
future of electronic design automation: Challenges and opportunities. IEEE Transactions on Computer-Aided Design of Integrated
Circuits and Systems, 39(9), 2843-2856.
Verma, A., & Pedram, M. (2019). Power,
thermal, and reliability optimization in digital VLSI systems. IEEE Transactions on Computer-Aided Design of Integrated Circuits
and Systems, 38(1), 3-16.
Bhardwaj, S., Chakrabarti, C., & Cao,
Y. (2020). Deep learning for integrated circuit layout optimization: Status, challenges, and future directions. Journal of Electronic
Testing, 36(6), 633-647.
Jain, R., & Zhai, Q. (2021). AI and
EDA: A roadmap for the next decade. IEEE Design & Test, 38(4), 47-58.Liu, S., Yin, Y., Yu, M., & Hu, C. (2020). AI in physical
design: Recent advances, challenges, and opportunities. IEEE Transactions on Computer-Aided Design of Integrated Circuits and Systems,
39(8), 2629-2642.
Sun, X., Li, H., & Xu, Y. (2021). Designing
in the era of process technology co-optimization. Proceedings of the IEEE, 109(6), 1029-1051.
Semiconductor Industry Association - 2019
SIA Factbook - FINAL (semiconductors.org)
Creswell, J. W. (2013). Qualitative inquiry
and research design: Choosing among five approaches. Sage Publications.
Denzin, N. K., & Lincoln, Y. S. (Eds.).
(2011). The Sage handbook of qualitative research. Sage Publications.
Agrawal, A., Gans, J., & Goldfarb,
A. (2017). What to expect from artificial intelligence. MIT Sloan Magagement Review, 2.
Alagappan, G., Ong, J. R., Yang, Z.,
Ang, T. Y., Zhao, W., Jiang, Y., & Png, C. E. (2022). Leveraging AI in Photonics and Beyond. Photonics, 9, 75.
Alam, A. (2021). Should robots replace
teachers? Mobilisation of AI and learning analytics in education. 1–12.
Alexopoulos, C., Lachana, Z., Androutsopoulou,
A., Diamantopoulou, V., Charalabidis, Y., & Loutsaris, M. A. (2019). How machine learning is changing e-government.
354–363.
Alrubei, S. M., Ball, E., & Rigelsford,
J. M. (2022). A secure blockchain platform for supporting AI-enabled IoT applications at the Edge layer. IEEE Access, 10,
18583–18595.
Amrouch, H., Chowdhury, A. B., Jin, W.,
Karri, R., Khorrami, F., Krishnamurthy, P., & Tan, S. X. (2021). Special session: Machine learning for semiconductor test and
reliability. 2021 IEEE 39th VLSI Test Symposium (VTS, 1–11.
Amuru, D., Zahra, A., Vudumula,
H. V., Cherupally, P. K., Gurram, S. R., Ahmad, A., & Abbas, Z. (2023). AI/ML algorithms and applications in VLSI design and
technology. Integration.
Asadizanjani, N., Rahman, M. T., &
Tehranipoor, M. (2021). Physical Inspection of Integrated Circuits. In Physical Assurance (pp. 49–65). Springer.
Bailey, L. W. (2019). New technology
for the classroom: Mobile devices, artificial intelligence, tutoring systems, and robotics. In Educational Technology and the
new world of persistent learning (pp. 1–11). IGI Global.
Batra, G., Jacobson, Z., Madhav, S.,
Queirolo, A., & Santhanam, N. (2019). Artificial-intelligence hardware: New opportunities for semiconductor companies. McKinsey
and Company.
Berente, N. G., Recker, J., & Santhanam,
R. (2021). Managing artificial intelligence. MIS Quarterly, 45(3), 1433–1450.
Beres, R. N., Wang, X., Blaabjerg, F.,
Liserre, M., & Bak, C. L. (2015). Optimal design of high-order passive-damped filters for grid-connected applications. IEEE
Transactions on Power Electronics, 31(3), 2083–2098.
Bhunia, S., & Tehranipoor, M. (2018).
The Hardware Trojan War. Springer.
Cañamares, R., Castells, P., &
Moffat, A. (2020). Offline evaluation options for recommender systems. Information Retrieval Journal, 23(4), 387–410.
Chan, C. H., Cheng, L., Deng, W., Feng,
P., Geng, L., Huang, M., & Zhang, Z. (2022). Trending IC design directions in 2022. Journal of Semiconductors, 43,
071401–1.
Chaudhary, M. W., Heinig, A., & Choubey,
B. (2020). Energy-area aware channel design for multi-chip interfaces. 2020 IEEE 29th Conference on Electrical Performance of
Electronic Packaging and Systems (EPEPS, 1–3.
Chen, T. (2018). Anomaly detection
in semiconductor manufacturing through time series forecasting using neural networks [(Doctoral dissertation,]. Massachusetts
Institute of Technology.
Chen, T., Zhang, G. L., Yu, B., Li, B.,
& Schlichtmann, U. (2022). Machine Learning in Advanced IC Design: A Methodological Survey. Power, 55, 53.
Chêne, M., Larras, B., Frappé,
A., & Kaiser, A. (2022). Distributed Artificial Intelligence Integrated Circuits For Ultra-Low-Power Smart Sensors. In 16ème
Colloque National du GDR SOC2.
Chu, C., & Ozdal, M. (2018). Introduction
to the special section on advances in physical design automation. ACM Transactions on Design Automation of Electronic Systems
(TODAES, 23(4), 1–2.
Cieplucha, M. (2019). Metric-driven verification
methodology with regression management. Journal of Electronic Testing, 35(1), 101–110.
D’Agostino, D., & Cesini, D.
(2021). Heterogeneous computing for AI and big data in high energy physics. Frontiers in Big Data, 4, 652881.
Dauvergne, P. (2021). The globalization
of artificial intelligence: Consequences for the politics of environmentalism. Globalizations, 18(2), 285–299.
Davenport, T. H., & Ronanki, R. (2018).
Artificial intelligence for the real world. Harvard Business Review, 96(1), 108–116.
Devi, S., Tilwankar, G., & Zele,
R. (2021). Automated Design of Analog Circuits using Machine Learning Techniques. 2021 25th International Symposium on VLSI
Design and Test (VDAT, 1–6.
Dhar, T., Kunal, K., Li, Y., Madhusudan,
M., Poojary, J., Sharma, A. K., & Sapatnekar, S. S. (2020). ALIGN: A system for automating analog layout. IEEE Design &
Test, 38(2), 8–18.
Dinu, A., Danciu, G. M., & Ogrutan,
P. L. (2022). Cost-efficient approaches for fulfillment of functional coverage during verification of digital designs. Micromachines,
13(5), 691.
Dinu, A., & Ogrutan, P. L. (2019).
Opportunities of using artificial intelligence in hardware verification. 2019 IEEE 25th International Symposium for Design and
Technology in Electronic Packaging (SIITME, 224–227.
Dong, C., He, G., Liu, X., Yang, Y.,
& Guo, W. (2019). A multi-layer hardware trojan protection framework for IoT chips. IEEE Access, 7, 23628–23639.
Doyle, L., McCabe, C., Keogh, B., Brady,
A., & McCann, M. (2020). An overview of the qualitative descriptive design within nursing research. Journal of Research
in Nursing, 25(5), 443–455.
Fan, A., Wang, J., & Aptekar, V.
(2020). Advanced Circuit Verification for Robust Design. 2020 IEEE 15th International Conference on Solid-State & Integrated
Circuit Technology (ICSICT, 1–3.
Fayazi, M., Colter, Z., Afshari, E.,
& Dreslinski, R. (2021). Applications of artificial intelligence on the modeling and optimization for analog and mixed-signal
circuits: A review. IEEE Transactions on Circuits and Systems I: Regular Papers, 68(6), 2418–2431.
Foxwell, H. J., & Foxwell, H. J.
(2020). Cleaning Your Data. Creating Good Data: A Guide to Dataset Structure and Data Representation, 75–91.
Fujita, M. (2019). 17.1 AI x Robotics:
Technology Challenges and Opportunities in Sensors, Actuators, and Integrated Circuits. 276–278.
Gaur, P., Rout, S. S., & Deb, S.
(2019). Efficient Hardware Verification Using Machine Learning Approach. 2019 IEEE International Symposium on Smart Electronic
Systems (ISES)(Formerly INiS, 168–171.
Greenstein, S. (2021). Economic Dependencies
in Integrated Circuits. IEEE Micro, 41(5), 130–132.
Greenstein, S. (2021). Shortages of integrated
circuits. IEEE Micro, 41(4), 86–88.
Gubbi, K. I., Beheshti-Shirazi, S. A.,
Sheaves, T., Salehi, S. P., Rafatirad, S., & Homayoun, H. (2022). Survey of Machine Learning for Electronic Design Automation.
Proceedings of the Great Lakes Symposium on VLSI 2022, 513–518.
Hampton, P. (2020). Keeping a secure
hold on data through modern electronic content management. Network Security, 2020(6), 8–11.
Hao, Y., Xiang, S., Han, G., Zhang, J.,
Ma, X., Zhu, Z., & Li, M. (2021). Recent progress of integrated circuits and optoelectronic chips. Science China Information
Sciences, 64(10), 1–33.
Hassani, H., Silva, E. S., Unger, S.,
TajMazinani, M., & Mac Feely, S. (2020). Artificial intelligence (AI) or intelligence augmentation (IA): What is the future?
Ai, 1(2), 8.
He, Y. (2021). Application of Artificial
Intelligence in Integrated Circuits. Journal of Physics: Conference Series, 12090.
Hoque, T., Slpsk, P., & Bhunia, S.
(2020). Trust issues in microelectronics: The concerns and the countermeasures. IEEE Consumer Electronics Magazine, 9(6),
72–83.
Huang, G., Hu, J., He, Y., Liu, J., Ma,
M., Shen, Z., & Wang, Y. (2021). Machine learning for electronic design automation: A survey. ACM Transactions on Design
Automation of Electronic Systems (TODAES, 26(5), 1–46.
Ilagan, L., Concepcion, R., Cabatuan,
M., & Roque, C. R. (2020). A 90 nm Static CMOS Technology Gate-Level Integrated Circuit Layout Classification and Error Detection
Using EfficientNet Model. 2020 IEEE 12th International Conference on Humanoid, Nanotechnology, Information Technology, Communication
and Control, Environment, and Management (HNICEM, 1–6.
Indrayadi, T. (2020). How to Select Participants
in My Research Study?: Sampling in Quasi-Experiment Research. Sampling in Quasi-Experiment Research (April 20, 2021). IJournals:
International Journal of Social Relevance & Concern, 8(5).
Islam, R. (2022). Feasibility Prediction
for Rapid IC Design Space Exploration. Electronics, 11(7), 1161.
James, A., Seth, A., Mukhopadhyay, S.
C., James, A., Seth, A., & Mukhopadhyay, S. C. (2022). Programming Raspberry Pi for IoT System. IoT System Design: Project
Based Approach, 51–79.
Jiahe, L. (2020). Machine Learning Aided
Design Optimization for Micro-chip Reliability Improvement. 2020 3rd World Conference on Mechanical Engineering and Intelligent
Manufacturing (WCMEIM, 131–135.
Kahng, A. B. (2018a). New directions
for learning-based IC design tools and methodologies. 2018 23rd Asia and South Pacific Design Automation Conference (ASP-DAC,
405–410.
Kahng, A. B. (2018b). New directions
for learning-based IC design tools and methodologies. 2018 23rd Asia and South Pacific Design Automation Conference (ASP-DAC,
405–410.
Kamal, N., Sarker, F., Mukta, M. S. H.,
& Mamun, K. A. (2020). Predictive Analysis of the Effects of Personality Traits on an Academic Program. 168–172.
Kaur, A., & Gill, S. S. (2016). Hybrid
swarm intelligence for VLSI floorplan. 2016 International Conference on Computing, Communication and Automation (ICCCA,
224–229.
Khailany, B. R., & Dally, W. J. (2020).
Accelerating chip design with machine learning. IEEE Micro, 40(6), 23–32.
Khakurel, J., Penzenstadler, B., Porras,
J., Knutas, A., & Zhang, W. (2018). The rise of artificial intelligence under the lens of sustainability. Technologies,
6(4), 100.
Khalid, F., Hasan, S. R., Zia, S., Hasan,
O., Awwad, F., & Shafique, M. (2020). Macler: Machine learning-based runtime hardware trojan detection in resource-constrained
iot edge devices. IEEE Transactions.
Kim, J. H. (2019). Multicollinearity
and misleading statistical results. Korean Journal of Anesthesiology, 72(6), 558–569.
Kim, T. T., Kim, B., Kim, J. Y., &
Kulkarni, J. P. (2022). Guest Editorial Revolution of AI and Machine Learning With Processing-in-Memory (PIM): From Systems, Architectures,
to Circuits. IEEE Journal on Emerging and Selected Topics in Circuits and Systems, 12(2), 333–337.
Kimura, A., Scholl, J., Schaffranek,
J., Sutter, M., Elliott, A., Strizich, M., & Via, G. D. (2020). A decomposition workflow for integrated circuit verification
and validation. Journal of Hardware and Systems Security, 4(1), 34–43.
Kish, F., Lal, V., Evans, P., Corzine,
S. W., Ziari, M., Butrie, T., & Welch, D. (2018). System-on-Chip Photonic Integrated Circuits. IEEE Journal of Selected
Topics in Quantum Electronics, 24(1), 1–20. https://doi.org/10.1109/jstqe.2017.2717863
Kline, D., Parshook, N., Johnson, A.,
Stine, J. E., Stanchina, W., Brunvand, E., & Jones, A. K. (2017). Sustainable IC design and fabrication. 2017 Eighth International
Green and Sustainable Computing Conference (IGSC, 1–8.
Klinefelter, A. (2020). How Software
Can” Chip In” to the IC Design Process: A Multidisciplinary Approach May Attract New Talent and Accelerate Innovation.
IEEE Solid-State Circuits Magazine, 12(4), 48–55.
Kovacs, I., Ţopa, M., Ene, M., Buzo,
A., & Pelz, G. (2020). A Metamodel Residual-based Stopping Criterion for Adaptive Verification of Integrated Circuits.
169–173.
Kulkarni, J. P. (2020). Chip Design 2020.
IEEE Micro, 40(6), 6–7.
Kumar, N., Kharkwal, N., Kohli, R., &
Choudhary, S. (2016). Ethical aspects and future of artificial intelligence. 2016 International Conference on Innovation and
Challenges in Cyber Security (ICICCS-INBUSH, 111–114.
Kyngäs, H., Kääriäinen,
M., & Elo, S. (2020a). The trustworthiness of content analysis. The Application of Content Analysis in Nursing Science Research,
41–48.
Kyngäs, H., Kääriäinen,
M., & Elo, S. (2020b). The trustworthiness of content analysis. The Application of Content Analysis in Nursing Science Research,
41–48.
Lee, H. (2022). The AI Shape and Post-Human
in Cultural Content. Specialusis Ugdymas, 1(43), 8675–8686.
Lee, J., Kim, D., & Lee, S. (2021).
An analysis of the Impact of AI Maker Coding Education on Improving Computing Thinking. Journal of The Korean Association of
Information Education, 25(5), 779–790.
Li, B., & Franzon, P. D. (2016).
Machine learning in physical design. 2016 IEEE 25th Conference on Electrical Performance of Electronic Packaging and Systems
(EPEPS, 147–150.
Li, B., Gu, J., & Jiang,
W. (2019). Artificial intelligence (AI) chip technology review. 114–117.
Li, E., Zeng, L., Zhou, Z., & Chen,
X. (2019). Edge AI: On-demand accelerating deep neural network inference via edge computing. IEEE Transactions on Wireless Communications,
19(1), 447–457.
Li, W., Wen, H., & Duan, P. (2022).
Key technologies and international trends in EDA field of digital IC design: A patent analysis. SHS Web of Conferences. 140.
Liang, B. S. (2021). Entrepreneurship-driven
growth in the integrated circuit design industry. Nature Electronics, 4(4), 234–236.
Lin, S. (2021). Application of Computer
Artificial Intelligence in Circuit Logic Simulation. Journal of Physics: Conference Series, 012071.
Liu, M., & Chakrabarty, K. (2021).
Adaptive Methods for Machine Learning-Based Testing of Integrated Circuits and Boards. 2021 IEEE International Test Conference
(ITC, 153–162.
Liu, M., Yang, D., He, F., Li, M., &
Doss, D. A. (2016). Perspectives of technology and the instrumentalist paradigm. Allied Academies International Conference.
Academy of Organizational Culture, Communications and Conflict. Proceedings.21, 29.
Liu, W. K., Tan, B., Fung, J. M., Karri,
R., & Chakrabarty, K. (2022). Hardware-Supported Patching of Security Bugs in Hardware IP Blocks. IEEE Transactions on Computer-Aided
Design of Integrated Circuits and Systems.
Lodhi, F. K., Khalid, F., Osman Hasan,
F. A., & Hasan, S. R. (XXXX). A self-learning framework to detect the intruded integrated circuits. 2016 IEEE International
Symposium on Circuits and Systems (ISCAS, 1702–1705.
Lu, H., Li, Y., Chen, M., Kim, H., &
Serikawa, S. (2018). Brain intelligence: Go beyond artificial intelligence. Mobile Networks and Applications, 23(2),
368–375.
Manolache, C., Caranica, A., Stănescu,
M., Cucu, H., Buzo, A., Diaconu, C., & Pelz, G. (2022). Advanced Operating Conditions Search applied in Analog Circuit Verification.
1–4.
Marinova, G. I., & Bitri, A. K. (2021).
Challenges and opportunities for semiconductor and electronic design automation industry in post-Covid-19 years. 1208(1),
012036.
Martinez, S., & Valverde, J. C. (2022).
Influence of Context on Greatest Common Divisor Problem Solving: A Qualitative Study. Mathematics, 10(8), 1325.
Martins, V. A., Guex, J. P., Chau, W.
J., & Oliveira, M. H. (2017). Verification Challenges in Analog-Digital Interfaces. In Seminatec 2017 XII Workshop on Semiconductors
and Micro & Nano Technology (pp. 1–2).
Matsui, H., Takeda, Y., & Tokito,
S. (2019). Flexible and printed organic transistors: From materials to integrated circuits. Organic Electronics, 75,
105432.
Miceli, A., Yoshii, K., & Foster,
I. T. (2022). Pushing compute and AI onto detector silicon. In Instrumentation and Detectors (pp. 1–2).
Mina, R., Jabbour, C., & Sakr, G.
E. (2022). A Review of Machine Learning Techniques in Analog Integrated Circuit Design Automation. Electronics, 11(3),
435.
Mirabbasi, S., Fujino, L. C., & Smith,
K. C. (2023). Through the Looking Glass—The 2023 Edition: Trends in solid-state circuits from ISSCC. IEEE Solid-State
Circuits Magazine, 15(1), 45–62.
Miranda, B. (2020). Sketching: A Cognitively
inspired Compositional Theorem Prover that Learns to Prove-a Proposal. Illinois Library, 1.
Mittelsteadt, M. (2021). AI Verification.
Center for Security and Emerging Technology.
Moness, M., Gaber, L., Hussein, A. I.,
& Ali, H. M. (2022). Automated Design Error Debugging of Digital VLSI Circuits. Journal of Electronic Testing, 38(4),
395–417.
Monroe, D. (2018). Chips for artificial
intelligence. Communications of the ACM, 61(4), 15–17.
Moradi, R., Nezamabadi-pour, H., &
Soleimanpour, M. (2019). Modified distributed Bee algorithm in task allocation of swarm robotic. 755–758.
Müller, V. C., & Bostrom, N.
(2016). Future progress in artificial intelligence: A survey of expert opinion. In Fundamental issues of artificial intelligence
(pp. 555–572). Springer.
Myny, K. (2018). The development of flexible
integrated circuits based on thin-film transistors. Nature Electronics, 1(1), 30–39.
Nardi, A., Camdzic, S., Armato, A., &
Lertora, F. (2019). Design-for-safety for automotive IC design: Challenges and opportunities. 2019 IEEE Custom Integrated Circuits
Conference (CICC, 1–8.
Naswali, E., Quiros, A. C., & Chandran,
P. (2019). DNNLibGen: Deep neural network based fast library generator. 2019 26th IEEE International Conference on Electronics,
Circuits and Systems (ICECS, 574–577.
Noble, H., & Heale, R. (2019). Triangulation
in research, with examples. Evidence-Based Nursing, 22(3), 67–68.
Obermayer, N., & Tóth, V.
E. (2021). The Impact of Emerging Technologies on Knowledge Management. 585–XXIII.
Obilisetty, S. (2018). Digital intelligence
and chip design. 2018 International Symposium on VLSI Design, Automation and Test (VLSI-DAT, 1–4.
Ogrutan, P. L., & Dinu, A. (2019).
Opportunities of using artificial intelligence in hardware verification. 2019 IEEE 25th International Symposium for Design and
Technology in Electronic Packaging (SIITME, 224–227.
O’Regan, G. (2018). Integrated
Circuit. In The Innovation in Computing Companion (pp. 155–159).
O’Regan, G. (2021). Integrated
Circuit and Silicon Valley. In A Brief History of Computing (pp. 89–96). Springer.
Park, I., & Kim, S. (2020). Performance
indicator survey for object detection. 284–288.
Park, S. J., Bae, B., & J, K. (2017).
Application of machine learning for optimization of 3-D integrated circuits and systems system. IEEE Transactions on Very Large
Scale Integration (VLSI, 25(6), 1856–1865.
Pheng, M. S., & David, L. G. (2022).
Artificial Intelligence in Back-End Semiconductor Manufacturing: A Case Study. 2022 IEEE International Conference on Distributed
Computing and Electrical Circuits and Electronics (ICDCECE, 1–4.
Porrasmaa, S. (2021). Programmatic
Integrated Circuit Design in Context of Analog-to-Digital Converters. Aaltodoc.
Powe II, J. A. (2019). Qualitative
Study Exploring Engineering Personnel’s Perceptions of New Technology Integration in the Engineering Sector.
Principe, E. L., Asadizanjani, N., Forte,
D., Tehranipoor, M., Chivas, R., DiBattista, M., & Mastovich, J. (2017). Steps toward automated deprocessing of integrated
circuits. ISTFA 2017: Proceedings from the 43rd International Symposium for Testing and Failure Analysis, 285.
Riazi, M., Ghanbar, H., & Rezvani,
R. (2023). Qualitative data coding and analysis: A systematic review of the papers published in the Journal of Second Language
Writing. Iranian Journal of Language Teaching Research, 11(1), 25–47.
Richards, K. A. R., & Hemphill, M.
A. (2018). A practical guide to collaborative qualitative data analysis. Journal of Teaching in Physical Education, 37(2),
225–231.
Richelli, A. (2021). Low-Voltage Integrated
Circuits Design and Application. Electronics, 10(1), 89.Li, B., Gu, J., & Jiang, W. (2019). Artificial intelligence
(AI) chip technology review. 114–117.
Saito, A., Takai, N., & Konno, S.
(2020). Determination of circuit topology and element values from desired characteristics by machine learning. 2020 27th IEEE
International Conference on Electronics, Circuits and Systems (ICECS, 1–4.
Sarma, K., & Vardhan, R. V. (2018).
Multivariate statistics made simple: A practical approach. Chapman and Hall/CRC.
Scheible, J., & Lienig, J. (2015).
Automation of analog IC layout: Challenges and solutions. Proceedings of the 2015 Symposium on International Symposium on Physical
Design, 33–40.
Schober, P., & Vetter, T. R. (2021).
Logistic regression in medical research. Anesthesia and Analgesia, 132(2), 365.
Self, C. C., & Roberts, C. (2019).
Credibility. In An integrated approach to communication theory and research (pp. 435–446). Routledge.
Seshia, S. A., Sadigh, D., & Sastry,
S. S. (2022). Toward verified artificial intelligence. Communications of the ACM, 65(7), 46–55.
Shaheen, M., & Pradhan, S. (2019).
Sampling in qualitative research. In Qualitative techniques for workplace data analysis (pp. 25–51). IGI Global.
Shanbhag, N. R., & Roy, S. K. (2022).
Comprehending In-memory Computing Trends via Proper Benchmarking. 2022 IEEE Custom Integrated Circuits Conference (CICC,
1–7.
Sharma, R., Rathor, V. S., Sharma, G.
K., & Pattanaik, M. (2021). A new hardware Trojan detection technique using deep convolutional neural network. Integration,
79, 1–11.
Shastri, B. J., Tait, A. N., Lima, T.,
Pernice, W. H., Bhaskaran, H., Wright, C. D., & Prucnal, P. R. (2021). Photonics for artificial intelligence and neuromorphic
computing. Nature Photonics, 15(2), 102–114.
Shi, G. (2018). Toward automated reasoning
for analog IC design by symbolic computation–A survey. Integration, 60, 117–131.
Shiraz, S., & Hasan, O. (2017). A
library for combinational circuit verification using the HOL theorem prover. IEEE Transactions on Computer-Aided Design of Integrated
Circuits and Systems, 37(2), 512–516.
Shoniker, M., Oleynikov, O., Cockburn,
B. F., Han, J., Rana, M., & Pedrycz, W. (2017). Automatic selection of process corner simulations for faster design verification.
IEEE Transactions on Computer-Aided Design of Integrated Circuits and Systems, 37(6).
Simatic, J., Cherkaoui, A., Bertrand,
F., Bastos, R. P., & Fesquet, L. (2017). A practical framework for specification, verification, and design of self-timed pipelines.
2017 23rd IEEE International Symposium on Asynchronous Circuits and Systems (ASYNC, 65–72.
Sims, J. M. (2010). A brief review of
the Belmont report. Dimensions of Critical Care Nursing, 29(4), 173–174.
Sparrow, R., & Hatherley, J. (2020).
High hopes for “Deep Medicine”? AI, economics, and the future of care. Hastings Center Report, 50(1),
14–17.
Sreenivasan, S. V. (2017). Nanoimprint
lithography steppers for volume fabrication of leading-edge semiconductor integrated circuits. Microsystems & Nanoengineering,
3(1), 1–19.
Srinivasulu, A., & Ravariu, C. (2020).
Emerging Artificial Intelligence Devices and The Underlying Technology. 2020 International Conference on Emerging Trends in
Communication, Control and Computing (ICONC3, 1–4.
Stempkovskiy, A., Telpukhov, D., &
Soloviev, R. (2017). Accurate method for identical fault search in logical circuits. 2017 IEEE East-West Design & Test Symposium
(EWDTS, 1–5.
Stenfors, T., Kajamaa, A., & Bennett,
D. (2020). How to… assess the quality of qualitative research. The Clinical Teacher, 17(6), 596–599.
Stopjakova, V., Rakus, M., Kovac, M.,
Arbet, D., Nagy, L., Sovcik, M., & Potocny, M. (2018). Ultra-low voltage analog ic design: Challenges, methods and examples.
Radioengineering, 27(1), 171–185.
Stratigopoulos, H. G. (2018). Machine
learning applications in IC testing. 2018 IEEE 23rd European Test Symposium (ETS, 1–10.
Sumrin, S., & Gupta, S. (2021). Establishing
validity and reliability in case study research projects. In The Routledge Companion to Marketing Research (pp. 119–131).
Routledge.
Sze, V., Chen, Y. H., Emer, J., Suleiman,
A., & Zhang, Z. (2017). Hardware for machine learning: Challenges and opportunities. 2017 IEEE Custom Integrated Circuits
Conference (CICC, 1–8.
Takai, N. (2020). Realization of a design-less
system for analog integrated circuits. Impact, 2020(1), 9–11.
Tasker, T. J., & Cisneroz, A. (2019).
Open-ended questions in qualitative research. Curriculum & Teaching Dialogue, 21(1/2), 119–122.
Theofanidis, D., & Fountouki, A.
(2018). Limitations and delimitations in the research process. Perioperative Nursing-Quarterly Scientific, Online Official Journal
of GORNA, 7(3 September-December 2018), 155–163.
Vashistha, N., Lu, H. S., Woodard, D.
L., Asadizanjani, N., & Tehranipoor, M. M. (2021). Detecting Hardware Trojans Using Combined Self-Testing and Imaging. IEEE
Transactions on Computer-Aided Design of Integrated Circuits and Systems, 41(6), 1730–1743.
Vashistha, N., Rahman, M. T., Shen, H.,
Woodard, D. L., Asadizanjani, N., & Tehranipoor, M. (2018). Detecting hardware trojans inserted by untrusted foundry using
physical inspection and advanced image processing. Journal of Hardware and Systems Security, 2(4), 333–344.
Wang, L., & Luo, M. (2019). Machine
learning applications and opportunities in IC design flow. 2019 International Symposium on VLSI Design, Automation and Test
(VLSI-DAT, 1–3.
Wells-Cornwall, O. G. (2021). Transformational
Leadership and Fiscal Sustainability in the Eastern Caribbean Currency Union.
Wu, J., Lin, X., Guo, Y., Liu, J., Fang,
L., Jiao, S., & Dai, Q. (2021). Analog optical computing for artificial intelligence. Engineering.
Xiu, L. (2019). Time Moore: Exploiting
Moore’s Law from the perspective of time. IEEE Solid-State Circuits Magazine, 11(1), 39–55.
Xu, X., & Zhang, J. (2020). Rethinking
FPGA security in the new era of artificial intelligence. 2020 21st International Symposium on Quality Electronic Design (ISQED,
46–51.
Yantır, H. E., Eltawil, A. M., &
Salama, K. N. (2022). A hardware/software co-design methodology for in-memory processors. Journal of Parallel and Distributed
Computing, 161, 63–71.
Youssef, A. A., Murmann, B., & Omran,
H. (2020). Analog IC design using precomputed lookup tables: Challenges and solutions. IEEE Access, 8, 134640–134652.
Zezin, D. (2022). Modern Open Source
IC Design tools for Electronics Engineer Education. 2022 VI International Conference on Information Technologies in Engineering
Education (Inforino, 1–4.
Appendix A: Questionnaire
Demographics
Age (Years) |
|
Gender |
Male |
Female |
Other |
Location |
|
Experience in Computing (Years) |
|
| 1. | How do you rate yourself on understanding AI? |
| | |
Very high |
High |
Neutral |
Low |
Very low |
| 2. | How do you rate yourself on understanding IC design? |
| | |
Very high |
High |
Neutral |
Low |
Very low |
AI & IC Design
| 3. | What level of disruption is likely to occur to IC design from AI? |
| | |
Very high |
High |
Neutral |
Low |
Very low |
| 4. | How will this disruption occur if you think it will happen? |
| 5. | How relevant is Moore’s law today? |
| | |
Very high |
High |
Neutral |
Low |
Very low |
| 6. | Is Moore’s law dead in your opinion? Why So? |
| 7. | What level is AI likely to influence IC design in the future? |
| | |
Very high |
High |
Neutral |
Low |
Very low |
| 8. | What is the likelihood of AI-based IC design overtaking engineer-based IC design? |
| | |
Very likely |
Likely |
Neutral |
Unlikely |
Very unlikely |
| 9. | How will IC design change over time in the coming future? |
| 10. | Do we currently need customized chips to fully support AI and ML? |
| | |
| | |
| 10.1 | Why so? (For your choice above) |
| 11. | How useful is the contribution of AI in developing better computer designs across: |
11.1 Custom chips?
Very high |
High |
Neutral |
Low |
Very low |
11.2 More powerful computers?
Very high |
High |
Neutral |
Low |
Very low |
| 12. | How likely is it for AI to provide solutions for IC design? |
| | |
Very likely |
Likely |
Neutral |
Unlikely |
Very unlikely |
| 13. | Do you have knowledge of the verification process of IC design? |
| | |
| 14. | How likely will AI help improve the verification process of IC design? |
| | |
Very likely |
Likely |
Neutral |
Unlikely |
Very unlikely |
| 15. | How likely are AI approaches to take over IC design verification? |
| | |
Very likely |
Likely |
Neutral |
Unlikely |
Very unlikely |
| 16. | In a few words, what is your opinion on AI improving IC design? |
| 17. | What are the key areas necessary to integrate AI automation in IC design? |
| 18. | How likely is it to keep advanced IC design costs low? |
| | |
Very likely |
Likely |
Neutral |
Unlikely |
Very unlikely |
18.1
How so? (Feedback)
| 19. | Do we generally need so much powerful computers? Why? |
| 20. | Do you have any other relevant information/insights about AI Technology involvement within future IC design? |
58
v3.23.2
X |
- DefinitionBoolean flag that is true when the XBRL content amends previously-filed or accepted submission.
+ References
+ Details
Name: |
dei_AmendmentFlag |
Namespace Prefix: |
dei_ |
Data Type: |
xbrli:booleanItemType |
Balance Type: |
na |
Period Type: |
duration |
|
X |
- DefinitionFor the EDGAR submission types of Form 8-K: the date of the report, the date of the earliest event reported; for the EDGAR submission types of Form N-1A: the filing date; for all other submission types: the end of the reporting or transition period. The format of the date is YYYY-MM-DD.
+ References
+ Details
Name: |
dei_DocumentPeriodEndDate |
Namespace Prefix: |
dei_ |
Data Type: |
xbrli:dateItemType |
Balance Type: |
na |
Period Type: |
duration |
|
X |
- DefinitionThe type of document being provided (such as 10-K, 10-Q, 485BPOS, etc). The document type is limited to the same value as the supporting SEC submission type, or the word 'Other'.
+ References
+ Details
Name: |
dei_DocumentType |
Namespace Prefix: |
dei_ |
Data Type: |
dei:submissionTypeItemType |
Balance Type: |
na |
Period Type: |
duration |
|
X |
- DefinitionAddress Line 1 such as Attn, Building Name, Street Name
+ References
+ Details
Name: |
dei_EntityAddressAddressLine1 |
Namespace Prefix: |
dei_ |
Data Type: |
xbrli:normalizedStringItemType |
Balance Type: |
na |
Period Type: |
duration |
|
X |
- DefinitionAddress Line 2 such as Street or Suite number
+ References
+ Details
Name: |
dei_EntityAddressAddressLine2 |
Namespace Prefix: |
dei_ |
Data Type: |
xbrli:normalizedStringItemType |
Balance Type: |
na |
Period Type: |
duration |
|
X |
- Definition
+ References
+ Details
Name: |
dei_EntityAddressCityOrTown |
Namespace Prefix: |
dei_ |
Data Type: |
xbrli:normalizedStringItemType |
Balance Type: |
na |
Period Type: |
duration |
|
X |
- DefinitionCode for the postal or zip code
+ References
+ Details
Name: |
dei_EntityAddressPostalZipCode |
Namespace Prefix: |
dei_ |
Data Type: |
xbrli:normalizedStringItemType |
Balance Type: |
na |
Period Type: |
duration |
|
X |
- DefinitionName of the state or province.
+ References
+ Details
Name: |
dei_EntityAddressStateOrProvince |
Namespace Prefix: |
dei_ |
Data Type: |
dei:stateOrProvinceItemType |
Balance Type: |
na |
Period Type: |
duration |
|
X |
- DefinitionA unique 10-digit SEC-issued value to identify entities that have filed disclosures with the SEC. It is commonly abbreviated as CIK.
+ ReferencesReference 1: http://www.xbrl.org/2003/role/presentationRef -Publisher SEC -Name Exchange Act -Number 240 -Section 12 -Subsection b-2
+ Details
Name: |
dei_EntityCentralIndexKey |
Namespace Prefix: |
dei_ |
Data Type: |
dei:centralIndexKeyItemType |
Balance Type: |
na |
Period Type: |
duration |
|
X |
- DefinitionIndicate if registrant meets the emerging growth company criteria.
+ ReferencesReference 1: http://www.xbrl.org/2003/role/presentationRef -Publisher SEC -Name Exchange Act -Number 240 -Section 12 -Subsection b-2
+ Details
Name: |
dei_EntityEmergingGrowthCompany |
Namespace Prefix: |
dei_ |
Data Type: |
xbrli:booleanItemType |
Balance Type: |
na |
Period Type: |
duration |
|
X |
- DefinitionCommission file number. The field allows up to 17 characters. The prefix may contain 1-3 digits, the sequence number may contain 1-8 digits, the optional suffix may contain 1-4 characters, and the fields are separated with a hyphen.
+ References
+ Details
Name: |
dei_EntityFileNumber |
Namespace Prefix: |
dei_ |
Data Type: |
dei:fileNumberItemType |
Balance Type: |
na |
Period Type: |
duration |
|
X |
- DefinitionTwo-character EDGAR code representing the state or country of incorporation.
+ References
+ Details
Name: |
dei_EntityIncorporationStateCountryCode |
Namespace Prefix: |
dei_ |
Data Type: |
dei:edgarStateCountryItemType |
Balance Type: |
na |
Period Type: |
duration |
|
X |
- DefinitionThe exact name of the entity filing the report as specified in its charter, which is required by forms filed with the SEC.
+ ReferencesReference 1: http://www.xbrl.org/2003/role/presentationRef -Publisher SEC -Name Exchange Act -Number 240 -Section 12 -Subsection b-2
+ Details
Name: |
dei_EntityRegistrantName |
Namespace Prefix: |
dei_ |
Data Type: |
xbrli:normalizedStringItemType |
Balance Type: |
na |
Period Type: |
duration |
|
X |
- DefinitionThe Tax Identification Number (TIN), also known as an Employer Identification Number (EIN), is a unique 9-digit value assigned by the IRS.
+ ReferencesReference 1: http://www.xbrl.org/2003/role/presentationRef -Publisher SEC -Name Exchange Act -Number 240 -Section 12 -Subsection b-2
+ Details
Name: |
dei_EntityTaxIdentificationNumber |
Namespace Prefix: |
dei_ |
Data Type: |
dei:employerIdItemType |
Balance Type: |
na |
Period Type: |
duration |
|
X |
- DefinitionLocal phone number for entity.
+ References
+ Details
Name: |
dei_LocalPhoneNumber |
Namespace Prefix: |
dei_ |
Data Type: |
xbrli:normalizedStringItemType |
Balance Type: |
na |
Period Type: |
duration |
|
X |
- DefinitionBoolean flag that is true when the Form 8-K filing is intended to satisfy the filing obligation of the registrant as pre-commencement communications pursuant to Rule 13e-4(c) under the Exchange Act.
+ ReferencesReference 1: http://www.xbrl.org/2003/role/presentationRef -Publisher SEC -Name Exchange Act -Number 240 -Section 13e -Subsection 4c
+ Details
Name: |
dei_PreCommencementIssuerTenderOffer |
Namespace Prefix: |
dei_ |
Data Type: |
xbrli:booleanItemType |
Balance Type: |
na |
Period Type: |
duration |
|
X |
- DefinitionBoolean flag that is true when the Form 8-K filing is intended to satisfy the filing obligation of the registrant as pre-commencement communications pursuant to Rule 14d-2(b) under the Exchange Act.
+ ReferencesReference 1: http://www.xbrl.org/2003/role/presentationRef -Publisher SEC -Name Exchange Act -Number 240 -Section 14d -Subsection 2b
+ Details
Name: |
dei_PreCommencementTenderOffer |
Namespace Prefix: |
dei_ |
Data Type: |
xbrli:booleanItemType |
Balance Type: |
na |
Period Type: |
duration |
|
X |
- DefinitionTitle of a 12(b) registered security.
+ ReferencesReference 1: http://www.xbrl.org/2003/role/presentationRef -Publisher SEC -Name Exchange Act -Number 240 -Section 12 -Subsection b
+ Details
Name: |
dei_Security12bTitle |
Namespace Prefix: |
dei_ |
Data Type: |
dei:securityTitleItemType |
Balance Type: |
na |
Period Type: |
duration |
|
X |
- DefinitionBoolean flag that is true when the Form 8-K filing is intended to satisfy the filing obligation of the registrant as soliciting material pursuant to Rule 14a-12 under the Exchange Act.
+ ReferencesReference 1: http://www.xbrl.org/2003/role/presentationRef -Publisher SEC -Name Exchange Act -Section 14a -Number 240 -Subsection 12
+ Details
Name: |
dei_SolicitingMaterial |
Namespace Prefix: |
dei_ |
Data Type: |
xbrli:booleanItemType |
Balance Type: |
na |
Period Type: |
duration |
|
X |
- DefinitionBoolean flag that is true when the Form 8-K filing is intended to satisfy the filing obligation of the registrant as written communications pursuant to Rule 425 under the Securities Act.
+ ReferencesReference 1: http://www.xbrl.org/2003/role/presentationRef -Publisher SEC -Name Securities Act -Number 230 -Section 425
+ Details
Name: |
dei_WrittenCommunications |
Namespace Prefix: |
dei_ |
Data Type: |
xbrli:booleanItemType |
Balance Type: |
na |
Period Type: |
duration |
|
GBT Technologies (PK) (USOTC:GTCH)
Historical Stock Chart
From Jun 2024 to Jul 2024
GBT Technologies (PK) (USOTC:GTCH)
Historical Stock Chart
From Jul 2023 to Jul 2024